Statistical_Theory
Statistical_Theory
Friday, July 03, 2020
This is for the bottom of the 10th or later innings, with a runner placed on second base.

This is how to read each line:
- -1: the home (batting) team entered the bottom of the 10th+ down by 1 run
- Actual 10th: how actual MLB teams performed in the bottom of the 10th with a runner on 2B and 0 outs. Subject to small sample size at larger leads.
- Actual 9th (same link as above): same idea as above, except bottom of the 9th
- RE24: using actual MLB team run scoring patterns in a random inning, and applying it to the 9th inning (with runner on 2B and 0 outs). While this is NORMALLY what I prefer in any inning 1 through 8, when it comes to the 9th inning, with reliever usage and small-ball scenarios, I try not to use it. It’s a great baseline however
- PROB: using a simple probability model
If you need a really really quick shorthand:
- tied: 80%
- down 1: 40% (half of above)
- down 2: 20% (half of above)
- down 3: 10% (half of above)
- down 4: 5% (half of above)
- down 5: 2.5% (half of above)
So, just remember “80% chance of winning” for the home/batting team tied, and then keep dividing by 2 for each run.
Saturday, June 13, 2020
I posted on Twitter something that is common knowledge among the saber folk, the 10:1 runs:wins relationship.
What is somewhat common knowledge is the relationship of bases and outs to Runs scored. Bill James taught us that with Runs Created, as a function of OBP to SLG.
What might be less common knowledge is how wOBA fits into this. wOBA is scaled to OBP and is proportional to SLG. And therefore, wOBA squared is proportional to runs scored.
However, when we talk about individual players, we really prefer to report in terms of wOBA and not wOBA squared. That’s because, at least for hitters, their impact to a team follows a linear approach, not a squared approach. This is why Linear Weights, not (the basic version of) Runs Created is preferred. And this is why a Runs Created approach that goes through a “theoretical team approach” is preferred. In other words, we can apply the Runs Created concept, but with about 8/9ths of it being linear. I hope that made sense.
So, if we want to know about how talented a team of batters is, we’d average their wOBA, not their wOBA squared (aka Runs). At the individual game level, it gets even worse, because that squared approach will really make larger the impact than it is. In other words, there’s a certain level of “running up the score” because of the way baseball is built.
And so, I thought: why don’t we take the square root of the runs scored and runs allowed? And then take the difference? And wouldn’t you know it: it’s (slightly) better than taking the actual difference in runs scored. I looked at the 660 team-seasons since 1998: 371 teams were closer to their actual W/L record following the Square Root of Runs (Root Runs) approach, while 289 teams were closer using the straight Run Differential approach. That’s 56% to 44%, which is fairly resounding as far as these things go.
The one place I’d be a bit worried, but not too much, is how it relates to pitchers. Pitcher interact with themselves. And so, you DO want a Runs (or wOBA squared) approach. However, adding that up at the game level probably hurts more than it helps. In other words, things get exaggerated at the game level and so, it might still work out going with a wOBA (or Root Runs) approach.
Anything more, and that’s for aspiring saberists to tackle. Actually, the veteran saberists should as well. This is not as obvious as it looks.
Wednesday, February 26, 2020
Good work from Jim here, if you focus on the things he'd doing, and not jump to any overall conclusions. Think about the title of this thread: evaluating the PLAY v attributing the influence of the player on the play. It's going to explain why you see the results you see, and why you should be careful with the conclusions. I talk about this dozens of times, and it'll save you alot of head scratching if you can keep remembering this.
Eventually, once we roll out Layered Hit Probability, it will all make sense.
Thursday, February 06, 2020
This is an expansion of a twitter post I made, though it will not not be fully expanded. Indeed, I have no idea how long I will take to write this blog post, but I expect it will be less than ten minutes.
Bill James had an article a few years ago saying that you could summarize the history of the Roman Empire in one sentence. Or one paragraph. Or several paragraphs. Or a book. Or an encyclopedia(*). In other words, however deep you wanted to go into the abyss, we could go.
(*) Assuming you know what that is.
First thing you want to know is the distribution of the talent level of the teams. Only god knows. But, we can infer it based on observations. If we observe that the win% based on 162 games is one standard deviation of .072(**), then the TRUE distribution is .060. We get that as:
.072^2 = true^2 + random^2
Where random is .5/root(N), where N = 162, and .5 is the root of p*q, where p is the average win percentage of .5 and q is 1-p.
(**) which is the historical average at some point, and I don’t know what it is more recently, though it can’t be that much different if you look at it over a few years)
So, we can reasonably estimate that in MLB the true talent distribution at the team level is one SD = .060 (***). To figure out the difference in talent between two random teams in this distribution, it is simply root 2 times .060 or .085.
(***) Knowing that, you can ALSO estimate the talent distribution at the player level! That’s another blog entry.
Knowing the standard deviation is one thing. What we want to know is the average difference. And roughly speaking, that is about 80% of the standard deviation. So, .085 x 80%. Therefore, the average difference is just under .070. Then we have the home site advantage, which lets worse teams beat better teams (but also allows better teams to not let random variation beat them). In MLB, with the home site advantage at about 54%, it doesn’t really change much, pushing it above .070.
And so, in MLB, if you have two teams, and you KNOW which is the more talented team, then the more talented team will win 57% of the time. On average.
?Suppose you had a ranking of starting pitchers, and you wanted to convert that into "points". How would you do that? You might be tempted to look at your 150 SP and give 150 to the first place pitcher and 149 to the second, all the way down to 1 for the 150th. That would however imply that the value spacing between each pitcher matches exactly to the ranking spacing. But we all know the gap between 1 and 21 is much larger than the gap between 101 and 121.
So what I do is first have some idea as to what that spacing should be. And for that, I turn to Weighted Enhanced Game Score
?
(Click to embiggen)
On the left chart is all the data points of the pitchers, which you can see is decidely not linear. In fact, that line follows a log function of about: 65 minus 4 * ln(x). You might be afraid of that ln(x). On the right, I changed the x-axis from Ordinal Ranking (1 to 150) to the ln(Ordinal Ranking). ln(1) = 0 and ln(150) ~= 5. Once you see the data laid out like that, you can now see a pretty close to a straight line. In other words, to convert a non-straight line into a straight-line, you need to apply some function to your x-axis. In this case, ln(x). Other times you may need to apply an exponential, or a quadratic equation, etc. (Sometimes, you won't get so lucky.)
While this function is 65 minus 4*ln(x) as the best fit for the top 150 SP, the AVERAGE of that is 49. Therefore, since the only purpose of using Game Score here was to give me an idea as to the relationship, I can tweak it to 66 minus 4*ln(x) so that I can an average Game Score of 50. The nice property of ln(1) = 0 is that the intercept value (66 in this case) ends up being the value of my #1 guy. And a 66 Game Score for the top pitcher is about a reasonable number.
Thursday, December 05, 2019
When the Marlins made their comeback against the Cubs in the 2003 playoffs, I described how WPA worked on a play by play (and in the case of the fan, pitch by pitch) basis. A couple of weeks ago, I described Layered Hit Probability, all the various layers we have to go through in order to explain the how/why that a play happened.
?Sam Miller lays it all out with what we are up against if we try to go to the ultimate, and describe all the baserunning and fielding involved in a play. And he makes the salient point:
To give credit on all of them means building statistical systems that can make assumptions that hold true in as many cases as possible -- and that don't require hours (and that don't rely on personal opinions) for each of them.
What Sam did is identified what we call Action Events. At every Action Event, we stop the play, and understand the landscape. We identify what is the run potential (actually win potential) at that point in time. Then we fast forward to the next Action Event and ask the same question. And we capture that change, and assign that change to the change agent(s) between the two Action Events. And on and on we go, much like I described with the Marlins/Cubs, but far more in-depth, as Sam has done. With the key point that we make sure it all adds up, as Sam showed.
And once we have it all broken down for all plays in an inning or a game or a season, we can tally it all. You can see it in the Cubs/Marlins:
The tally:
Prior + SS = +.076
Prior + Alou = +.051
Remlinger = +.001
Remlinger + Fielders = -.016
Dusty = -.017
Fan = -.031
Prior = -.051
Gonzalez = -.184
Farns + OF = -.271
Prior + OF = -.476
Manager = -.017
Fan = -.031
Pitchers = -.368
Fielders = -.502
TOTAL: -.918 (.018 – .936 = .918)
And the kicker is going to be, that once we have a Statcast WAR, that we may be able to explain the PLAYS, we may be introducing a bunch of random variation into a PLAYER. We'll be taking three steps forward on explaining baseball, but we may be running in place in explaining a baseball player. This is why FIP has such a strong footprint, taking the bird's eye view in explaining a baseball player. You have to be careful in conflating the IDENTITY of the players involved in a play, with the INFLUENCE of the player (as opposed to the effect of random variation). And this gets into the bittersweet symphony of explaining baseball, which I tried to describe in this two-part thread from a while ago.
Wednesday, November 06, 2019
?I ran a series of polls of the Straight Arrow voters among the 9 player candidates, along with intermediary results, which are close to the current results. To read that, it says that if you were to select ONE player, and one player only, Lou Whitaker would get 34% (using that link) of the votes. It's currently at 35%, and I'll use the most current results for this blog post.
So, you can see that in a "must 1" balloting process, if the threshold is 75%, no one would get inducted. There's too much vote splitting. Ah, but what if it was a "must 2" balloting process? What if everyone had to select 2 players? Could we figure that out? Yes!
Warning, math ahead.
Let's start from the perspective of Lou Whitaker. In a must 1, we already know that 65% did not select him. Of those 65, 12 of those was Don Mattingly that was selected. So, if we look at the 8 remaining (so, Whitaker, and the other 7, not Mattingly), we take Whitaker's strength (353) and divide it by the remaining strength (1000 minus Mattingly's 119) to get 40.1%. In other words, if Mattingly is off the board, then Whitaker will appear on 40% of those ballots, as the 2nd candidate. We repeat this for each player, and Whitaker will appear from 40% to 36% as the second candidate.
And how often do each of those happen? Well, we weight it by the 1st ballot voting rates. For MAttingly that's 12% and for John and Murphy that's 10% each, and so on. And when we do that, we get 39% for Whitaker. In other words, given that Whitaker was NOT the first selection on the ballot, he will be the SECOND selection on the ballot 39% of the time. And since he was NOT on the first selection 65% of the time, we take 65% times 39% and we get 25%. Whitaker appears on 25% of the ballots as the #2 candidate. We already know he appears on 35% of the ballots as the #1 candidate. And so, Whitaker, in a must-2 selection process, will appear on 60% of the ballots.
Mattingly, who was 12% on a must-1 process, is now at 25% on a must-2 process. Garvey, 2.7% on a must-1 is now 6.1% on a must-2.
End of Math
Ok, so this is how it works. I will now turn it over to the aspiring saberists. First figure out the strength values. If you don't know how to do that, then just use what I posted on Twitter. Secondly, repeat what I did for a must-1 and must-2. And then show us must-3 and must-4 and must-5 and must-6.
You'll have plenty of fun if you are a math enthusiast.
Wednesday, October 09, 2019
?Mike's got you covered with a brilliant layout of the relay heard round the world.
The next step is to figure out if it was worth it to even test the Rays. And it was not.
First, let's lay out the numbers.
- Top of 4th
- 1 out
- 2b and 3b
- home team up by 3
Which means
- .738 home win expectancy if hold up
- .718 home win expectancy if safe
- .840 home win expectancy if out
Batting team gains .020 wins if safe, loses .102 if out
Breakeven: 84%
- In other words, if you are at least 90% sure the runner will be safe, you SEND him:
.718 x .90
+ .840 x .10
= .730
Home team (defense) win expectancy goes from .738 to .730 in this case
- And if you are at best 80% sure the runner will be safe, you HOLD him, otherwise:
.718 x .80
+ .840 x .20
= .742
Home team (defense) win expectancy goes from .738 to .742 if you send him when you are at best 80% sure.
Why the high breakeven?
The difference between this situation, and most others, is that you do NOT want to make an out at home with 0 or 1 outs. That's because of the power of the potential sac fly. So, that's why you have to be really really sure that the runner will be safe. At least 84% sure. That's Tim Raines trying to steal a base, that's the confidence you need.
And when a runner is thrown out by this much, you know that it was not an 84% chance of being safe. The only way for Altuve to be safe is if the relay was not perfect. And with two guys throwing, that probably sets it at 50% chance of that happening. With two outs, this is the ideal send. With 0 or 1 out, it is not.
?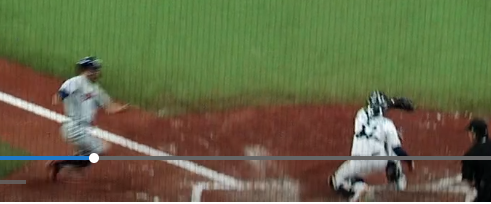
Monday, September 02, 2019
?About six months ago, in introducing a simple way to create the Catcher Framing metric, I also showed how to quickly test for park bias in that metric. It actually can apply to any metric. In any sport.
Let's apply this concept to the exit speed of a batted ball. The key to the concept is that we presume no relationship in talent between the home batters (and opposing pitchers) compared to the away batters (and home pitchers). What we do is for each park we figure the average exit speed for the home batters (or the bottom of the inning) and the away batters (or the top of the inning). In Fenway 2019 for example, the exit speed on the bottom of the inning was 90.7 mph (or +1.9 mph above league average) and in the top of the inning it was -0.1 mph from league average. We repeat this for all 30 parks, for the five years of Statcast.
If there is no correlation at all, and there shouldn't be based on our assumption of fact, we'll get an r close to 0. If we do get a larger correlation, that would point to some sort of park bias. That bias could be the tracking system. It could also be the players responding to the peculiarities of the park. And what do we get? r=0.06. In effect, an r close to 0, and therefore showing no park bias.
Aspiring saberists can use this technique, in any of the sports, to look for biases in metrics, whether measured like I am doing here, or calculated, as I did with the Catcher Framing.
?
Thursday, August 15, 2019
Darry Strawberry or Don Mattingly?
A few weeks ago, I ran a poll asking the style of player that fans preferred. Overwhelmingly, fans preferred Strawberry to Mattingly. Strawberry represents the three-true-outcome style (lots of HR, lots of walks, lots of strikeouts, not much in batting average). Mattingly is the opposite: a decent number of HR, not much walks, not much strikeouts, way high in batting average.
Overall, they had a quite similar effect on run generation. If you focus on their stats through their 20s, Mattingly came to bat 4851 times to Strawberry's 5137. So, a 286 PA advantage for Straw. Straw had 242 fewer hits, but 313 more walks, 111 more HR, and 827 more strikeouts. The rate stats tell the story more clearly as to their profile. Here are their BA, OBP, SLG
- .317 / .363 / .504 Mattingly
- .263 / .359 / .516 Strawberry
In other words, similar OBP, similar SLG, and a whopping difference in batting average. Is it better to have a low or high batting average?
Well, we can turn to wOBA. And Standard wOBA is .375 for Strawberry and .372 for Mattingly. In other words, the huge gap in batting average was inconsequential: we can describe their overall production (via wOBA) as being similar, which also matches their simlarity in OBP and SLG.
So, that let me to my poll question:
Which is the more productive hitter
A.
- .315 batting average
- .365 OBP
- .510 SLG
B.
- .260 batting average
- .365 OBP
- .510 SLG
Player A is the Mattingly, Dave Parker type. Fred Lynn and Jim Rice too if you wish.
Player B is the Strawberry, Mike Schmidt type. Eric Davis (without the speed) if you wish.
We can construct hitting lines of 700 PA as follows:
- Give the Mattingly type 650 AB, 50 walks, with 205 hits, 44 doubles, 4 triples, 25 HR. That's quintessential Mattingly. Quint Mattingly.
- Strawberry gets his 700 PA split as 601 AB, 99 walks, with 156 hits, 32 doubles, 4 triples, 37 HR. That's quintessential Strawberry. Quint Strawberry.
Those lines gives us identical OBP/SLG of .364/.511, which I am arguing (not really arguing, really stating as fact) is identical production, even if one guy has a .315 BA and the other has a .260 BA.
Indeed, their Standard wOBA is .379 for Quint Mattingly, and .378 for Quint Strawberry.
How does it happen that the tradeoff is basically even? Quint Mattingly has 49 more singles while Quint Strawberry has 49 more walks. In terms of run production, that gives Mattingly 7-8 more runs. Quint Mattingly has 12 more doubles to Quint Strawberry 12 more HR. That gives Strawberry 7-8 more runs.
In other words, giving away 49 singles for 49 walks is balanced by getting 12 more HR for 12 fewer doubles. Or if you wish -4 singles, -1 doubles = +4 walks, +1 HR. That's the tradeoff.
And that's why Quint Mattingly = Quint Strawberry. And that's why the batting average is inconsequential. And that the vast majority of voters used the higher batting average as essentially the tie-breaker is why we should stop talking about batting average. It's a bias that clouds our view of players.
Also: check out the take from Ben at Fangraphs.
Friday, July 12, 2019
I too thought it was ridiculous that one of out eight men thought they could.
But, the key is the competition setup. Is it a one-shot deal? Then, yes, that is totally laughable.
But, if this was a 2-set match? Things are different. This is where we rely on good luck. A game is made up of at least 4 points. (The way tennis is setup, you need to get 4 points and win by 2 in order to win a game.) You need to win 6 games to win a set, and two sets to win a match. And serves alternate.
So, for Serena to win the match on a shutout, she has to score 48 points to my zero. That would mean scoring 24 points by her serving, and she needs to score 24 points on my serve.
The only conceivable way for Serena to not score on her own serve is for her to double-fault. The chance that she would do that against me is probably 1 in a thousand. Or 99.9% she won't double-fault on any serve. So, .999^24 she won't double-fault, or 97.6%.
What is the chance she won't return my 24 serves? Let's see, she'll get 12 points simply because I'll double-fault. In the next 11 serves, she'll hit them back 99.99% of the time. And on the last serve, I'll Nick Kyrgios an underhand serve. She'll return that one 99% of the time.
So, she'll return my serve .9999^11 x .99 = 98.9%.
And 97.6% x 98.9% = 96.5% chance that Serena will get a shutout.
So, I think I have a 3.5% chance of scoring one point, if I'm given 48 opportunities to do so. That's 28:1 odds.
This would mean that I would be willing to bet 1000$, with the chance of winning 28,000$. And I think there's no way I would do that. You can do all the math I did, but the reality is that if I'm laying out 1000$ that I can get one point out of 48 tries on Serena, I'd expect at least a 100,000$ payout. That's 100:1 odds. That means I really have a 1% chance of getting a point. And I think that's probably being optimistic.
Saturday, July 06, 2019
?Something interesting happens with sports: opportunities are NOT handed out randomly. This little quirk actually is fairly critical. You see, the way Regression Toward The Mean (or the better term, Reversion To Form) works is that you need to know the population mean. But, since most of the playing time is given out to the better players, those players get more weight when you calculate the league average mean. What we actually want is the unweighted average of our population.
Something interesting happens when you do that. The classic way is to treat the league average mean as the population mean. And so, you would provide 200-300 PA of "Ballast" for your prior, the amount of weight to the population mean, to add to your observations for each player, to come up with the posterior, the True Talent Level of each player.
But, if instead of the actual league average (which is overweighted to the better players) you had the simple league average, an unweighted population mean, the amount of ballast is going to shrink considerably, probably under 100 PA. The population mean will also go down. Instead of say a .320 wOBA, it'll go down to say .300, maybe even lower. For guys with 700 PA, things won't change much. For example, .400 wOBA observed on 700 PA will give you a True Talent estimate of .379 with 250 PA of .320 wOBA ballast. But with 100 PA of .290 wOBA ballast, it's a .386 estimate.
Where it's REALLY going to matter is with guys with few PA. Those guys with the classic method would give you .320. And with this new method will give us .290. And naturally, the newer method is better. After all, the reason they have so few PA is BECAUSE we know they are below average hitters. We can't just ignore that critical piece of information.
So, this is for aspiring saberists to focus on this framework to come up with the better estimates, the better process, than just to rely on the league mean to represent the population mean.
***
This is also the idea behind the WARcels by the way. If you think about what I did, and why I did what I did, you will see that I did apply regression, but I did NOT use league average. I in fact, implicitly, used the replacement level. The true population mean is going to be somewhere between the replacement level and the weighted league average mean.
?The always fantastic Probability Jock Kincaid gives us a primer, with carefully constructed scenarios. You can especially see the care he takes when he notes that one SD = .072 in talent using historical data, then switches to one SD = .060 for the more recent decades.
Anyway, I loved the way he started, by going with purely unweighted coin to totally weighted, then choosing in-between.
Monday, June 03, 2019
Ballast
?Regression Toward The Mean (RTTM) is an important concept, a critical concept. But boy what a terrible name. Michael Lopez proposed Reversion To Form, which is a definite improvement. Regression has its own non-statistical definition, while Reversion really is about resetting the expectation from the observation. And To Form, as opposed to Toward The Mean is also better, as RTTM makes it seem that the player's talent is changing toward the population mean. Reversion To Form is really about setting our expectation of his talent level given the observation.
Bill James since the 1980s (and I can attest to this, since I remember everything he wrote, and I started reading him in the 1980s) has always used the term Ballast. In a nod to his brilliance, he knew he needed to do something, without the concept of Bayes being at the forefront. Which is why Bayes is beautiful, because we all use it, even without formality.
Priors
In order to establish the true rate of something based on the observation of that thing, we need to know something about the population that this thing was drawn from. This is called your Prior Distribution. The Prior requires both the mean and an amount. For something like OBP, the mean is the league mean, say .330, but more importantly is the regression amount. This is what Bill James calls Ballast. It's how much your observation of a player needs to be pulled toward the population of all players. For OBP, historically, it's in the 200-300 PA range. For something like K/PA, it's much lower, while for something like BABIP, it's much higher. In other words, the amount of Ballast you need is linked to how much that observation tells you about the player.
In sports, the skill that requires the least amount of Ballast is free throw shooting. As a general rule, the less "layers" there is between the physical effort required, and the end-result, the less Ballast you need. For free throws, there's the player at the free throw line, and the basket. There's no defense, there's no varying distance. In addition, because players are not chosen on their free throw skills (think Shaq), there's a naturally wide talent base to choose from. The wider the talent base, the less Ballast needed as well.
For things like BABIP, it's a crucial skill for a pitcher, so they are selected for it. (If a pitcher is hit too hard, he won't make it to MLB.) So, we already have a tight range. But in addition to that, you have the batter, the park, and the fielders. There's alot of layers to get through from pitcher physical skill to outcome. We need alot of Ballast.
Swing Rates
How about swing rates? We break up the area at the plate into four regions:
- Heart of the Plate
- Shadow Zone
- Chase Region
- Waste Region
A hitter has a hitting approach. A hitter does not really change his hitting approach, since that hitting approach is what has brought him to MLB in the first place. However, he will tinker, and as the years go by, he will start to adapt. So, while we suspect we're going to need more Ballast than free throw shooting, we also think he won't need as much as with Strikeouts.
Heart of the Plate
Let's look at the data. For pitches in the Heart of the Plate, hitters will swing 70-75% of the time, with one standard deviation being about 6% (among our sample hitters, who averaged 480 PA). Technically, I should be using number of pitches, not PA, but PA is an easier standard if comparing to other skills. As it so happens, there's about a 1:1 relationship between number of pitches in Heart of Plate and number of PA.
Anyway, since one standard deviation is 2% and our observed is 6%, that gives us a z-score of close to 3. Our Reversion To Form (or Regression Toward The Mean) is 1/z^2, or close to 12%. The Ballast (or Regression Amount) is .12/.88*480 = 65. In other words, we need to add 65 PA of Ballast to an observed swing rate for pitches in Heart of the Plate.
Shadow, Chase, Waste
Shadow Zone also requires about 65 PA of Ballast. However, since there are 1.7 pitches per PA in the Shadow Zone, the amount of Ballast of Pitches is closer to 40 Pitches.
Chase Region requires close to 40 PA of BAllast, or about 45 Pitches of Ballast.
Waste Region is 45 PA of Ballast or 125 Pitches of Ballast. In other words, how a batter swings in the Waste Region is not as indicative of his approach in the other regions.
For the sake of simplicity, let's add 50 PA of Ballast for each Region.
Adjacent Regions
Now, we can also learn about each Region by looking at the other three regions. After all, how a hitter approach the Heart of the Plate can be informed by how he approaches the Shadow, Chase, and Waste regions.
As it turns out, the weighting is close to:
The other regions are more iffy. Guys like Votto, the less you Chase, the more you swing in the Heart. For guys like Baez, the more you Chase, the more you swing anywhere.
Repeating for the other three regions, and we have the following for Shadow:
- 20% Heart
- 50% Shadow
- 30% Chase
In other words, the surrounding regions inform alot, but Chase is more indicative of the approach to Shadow, than Heart.
Chase:
- 25% Shadow
- 50% Chase
- 25% Waste
Chase is fairly equally informed from the other two.
Waste:
So, there you have it. In order to establish the skill level of a hitter at swinging in each of the 4 regions, you apply a 50 PA Ballast, along with the weighting of the adjacent skills. The aspiring saberist can of course focus more on pitches than PA, and be a bit more rigid in their approach. And especially focusing on players who might have a new "established" change in hitting approach.
Thursday, May 16, 2019
?Here we go. If you want to see statistics being misapplied, look no further than what you will read about SAT.
Any adjustment made is going to be biased in some form or other. I'll make the comparison with hockey, so as to not offend anyone. At some point, and probably this is still true, half the first round picks were Europeans. But, much less than half of NHL players are Europeans. Why is that? Because the NHL goes after the best European players. Once you get down to the 3rd and 4th line players, there's a cost/benefit applied: what does it cost to scout and bring over a European player, when there's someone almost as good in Canada and USA? In other words, Europe sends over disproportionately their best players, compared to what Canada and USA sends. That means that the AVERAGE European player in the NHL would have to be better than the average Canadian or American player. It's a selection bias.
If you just look at the first wave of Russian players in the NHL, you'd think that Russia only had hall of fame caliber players. Mogilny, Bure, Federov... Larianov, Makarov, (Krutov), Fetisov, Kasatonov. Again, selection bias.
How do you know you don't have a selection bias? When the average of that class is the same as all the other classes. LHP v RHP? If you check, they will have the same ERA and same FIP. (I haven't checked in many many years, so if it is not true, then we have a market inefficiency.) MLB players born in California compared to NJ? They should have the same WAR per PA and WAR per IP (though Trout is going to break the rule, so be careful with sample size too). If not, there's a market inefficiency. (Or like NHL, a cost/benefit that crystallizes this inefficiency.)
So, when you look at the SAT, be very careful especially for selection bias.
Saturday, March 30, 2019
?Phil has an article on the subject. MGL also sent me results of his tests a few months ago. I enjoy math, and if it was socially acceptable I'd be ensconced in numbers. When it comes to this issue, it is not natural to me. So, I have to run simulations to understand this.
This is what I did: I have 21 players, 7 of whom have 100 plate appearances, 7 have 900 plate appearances, and 7 have 6400 plate appearances. They all have a true talent .330 OBP. What standard deviation do we observe? In my sims, I get .027. What should we expect? I'm not exactly sure. However, if I take the harmonic mean of 100, 900, 6400, I get 266. And if you had 266 PA of .330 OBP, one SD is .029. So, I think that checks out.
Notice that I equally weighted the 21 players. What if instead I weight the players by their number of PA? Naturally, this is going to be driven largely by the guys with 6400 PA. In my sim, one standard deviation is .009. What should we expect? I'm not exactly sure. However, if I take the classic binomial, one SD from random variation for the three groups are:
- .047 : 100 PA
- .016 : 900 PA
- .006 : 6400 PA
If I square those SD, multiply by the PA, sum, divide by the 7400 PA, then take the square root, I get .0095. So, I think that checks out too.
***
Now, assuming I'm understanding what I did, and that what I did is correct, then I much prefer method 2, even if both methods are justifiable. When it comes to both uneven playing time AND uneven talent, then I would want my talent weighted by their number of PA. So that if someone says "what's the spread in talent?", I'd want to answer it with respect to how they are used.
Saturday, March 02, 2019
?In his series of using Game Score, Bill James highlighted the case of Mike Mussina and Roger Clemens in 2001, both pitching for the Yankees. Across the board, Mussina had the better season, but Clemens won the Cy Young, in large part due to his 20-3 record, compared to Mussina's 17-11. Since they were on the same team, a priori we would presume similar context. And therefore one would need to conclude that Clemens being 5.5 "games ahead" over Mussina in the W-L record is a reflection of Clemens himself.
But, we understand better than that. We understand that the TEAM SEASONAL run support does not itself imply that this is what we'd observe over 33 or 34 starts. What we ultimately care about is not the PRIOR but the POSTERIOR.
What's the difference? a priori is knowledge-before-observation. a posteriori is knowledge-after-observation. So, "knowing nothing about nothing", our Prior in determining the run support for Clemens and Mussina is about 5 runs per start, since the Yankees scored 5 runs per game. Now the observation. Let's assume we don't know how many runs they scored exactly for Mussina and Clemens, and all we had was their W-L and ERA (or RA/9). A 20-3 record in 33 starts and 220 IP with a 3.84 RA/9 for Clemens would likely imply 6.8 runs support per 9 IP. That's our observation.
Our Posterior requires that we consider both our prior (the 5 runs of the Yankees for the season) and our observation (or the implication of the observation) of 6.8. Therefore, our posterior will be some combination of the two. Without trying to come up with the proper balance, let's just split the two and have our Posterior as 5.9. In other words, knowing the Yankees run support, and observing what the Yankees did specifically with Clemens, we estimate his run support at 5.9 runs per start.
Doing the same with Mussina, our implied observation is 4.1 runs per start, so our Posterior is 4.5 runs per start.
And what was it in fact? Clemens was 5.7, so our Posterior was pretty close. And Mussina was 4.2, also decent.
So, absent knowing their actual run support, what is our best approach to estimating their run support:
- using only their prior (all Yanks starters get 5 runs per start)
- using only the observation implied by their W-L and RA9 (Clemens gets 6.8 and Mussina gets 4.1)
- using their posterior (meaning prior and observation)
Clearly, it's the posterior. And in part 2, we'll look at the Bayesian BABIP. As soon as I write it.
Friday, February 08, 2019
?I recently tweeted this out:
So with 625 PA, 1SD is 14 units of wRC+ and 1 win of WAR
At 625 PA, its about 71 runs = 100 units of wRC+, so 14 units of wRC+ is 10 runs or 1 win
This is a good shorthand rule. But what about two extreme cases:
- A walk+HR heavy hitter, who is otherwise league average wOBA and OBP
- A .500+ OBP and wOBA
For the first case, the standard deviation increases by about 4%. In the second case, it increases by 30%, but 9% is attributed just on the increase in OBP, so that the "profile" impact is 21%. In other words, you can reasonably estimate the standard deviation using just OBP, with some adjustment based on the "profile" of the hitter, which will add a few percentage points for the most part, even for guys like Trout.
(For Trout specifically, the standard deviation is 11% wider using OBP, and 22% wider using wOBA, meaning 11% of that is because of his "profile".)
So, for a league average hitter, his WAR has one SD = 1 win. For a great hitter, with the same 625 PA, it would be 1.2 wins.
***
I also talked about uncertainty a few months ago, which is a good blog post to review.
Friday, February 01, 2019
In this post, Hareeb makes this observation:
As it turns out, properly removing park factor noise (wRC+) is more important than capturing sequencing (Runs Scored).
I never really thought about it, but it seems like an insightful observation. Could we have figured that out without doing a regression? Let's see. I've never done this before, so let's see where it takes us.
As Hareeb reminds us, high runs scored is based on:
- high offensive talent (think true talent wOBA + true talent baserunning)
- timing of good events
- run-friendly parks?
Which is the most impactful? We can try to make a decent estimate. Let's take them one at a time.
Spread in team talent (offense and defense)
- One standard deviation in win% is about .072, which means that we can infer that one SD is .060.
- And since offense = defense, then we can estimate that one SD of win% attributed to offense is 1/root2 of .060, or .042.
- And since 10 runs ~ 1 win, then one SD of true talent run scoring per game is 0.42
- So over 162 games, that's 1 SD = 68 runs of true talent (or 1 SD = 82 runs of observation)
Spread in sequencing
- Roughly speaking, one SD of random variation of wOBA over 162 games is: 0.5 x root(38PA x 162G) = 39, which we can scale to runs by x0.8 = 31 runs
- If we add the random variation of wOBA to the true talent of team, we get one SD = 74 (root of 68^2+31^2)
- We are still short 34 runs, which is probably the effect of sequencing. I don't necessarily like this "leftover" approach, but we just need a decent starting point
Spread in parks
- One SD in park factors is probably 5%, which means that with ~ 4.5 x 162 = 729 runs, 5% is 36 runs
Sooooo... spread in parks and spread in sequencing and spread in random variation are.... all about very similar, with parks taking the slight lead! At least using this approach.
Hareeb points out that:
- wOBA + minimizing park effect = wRC+
- wOBA + park + sequencing = Runs Scored
And since wRC+ beat out Runs Scored, that means neutralizing park effects has more impact than ignoring sequencing! A brilliant observation. And given my approach, I would have expected something pretty close to that (though not necessarily to that magnitude).
Fantastic, I learned something new!
Wednesday, January 16, 2019
?This. This is what it looks like. Terrific job by Phil.
Recent comments
Older comments
Page 2 of 150 pages < 1 2 3 4 > Last ›Complete Archive – By Category
Complete Archive – By Date
FORUM TOPICS
Jul 12 15:22 MarcelsApr 16 14:31 Pitch Count Estimators
Mar 12 16:30 Appendix to THE BOOK - THE GORY DETAILS
Jan 29 09:41 NFL Overtime Idea
Jan 22 14:48 Weighting Years for NFL Player Projections
Jan 21 09:18 positional runs in pythagenpat
Oct 20 15:57 DRS: FG vs. BB-Ref
Apr 12 09:43 What if baseball was like survivor? You are eliminated ...
Nov 24 09:57 Win Attribution to offense, pitching, and fielding at the game level (prototype method)
Jul 13 10:20 How to watch great past games without spoilers