Abstract
Land surface phenology (LSP), the study of seasonal dynamics of vegetated land surfaces from remote sensing, is a key indicator of global change, that both responds to and influences weather and climate. The effects of climatic changes on LSP depend on the relative importance of climatic constraints in specific regions—which are not well understood at global scale. Understanding the climatic constraints that underlie LSP is crucial for explaining climate change effects on global vegetation phenology.
We used a combination of modelled and remotely-sensed vegetation activity records to quantify the interplay of three climatic constraints on land surface phenology (namely minimum temperature, moisture availability, and photoperiod), as well as the dynamic nature of these constraints. Our study examined trends and the relative importance of the three constrains at the start and the end of the growing season over eight global environmental zones, for the past three decades.
Our analysis revealed widespread shifts in the relative importance of climatic constraints in the temperate and boreal biomes during the 1982–2011 period. These changes in the relative importance of the three climatic constraints, which ranged up to 8% since 1982 levels, varied with latitude and between start and end of the growing season. We found a reduced influence of minimum temperature on start and end of season in all environmental zones considered, with a biome-dependent effect on moisture and photoperiod constraints. For the end of season, we report that the influence of moisture has on average increased for both the temperate and boreal biomes over 8.99 million km2. A shifting relative importance of climatic constraints on LSP has implications both for understanding changes and for improving how they may be modelled at large scales.
Original content from this work may be used under the terms of the Creative Commons Attribution 3.0 licence.
Any further distribution of this work must maintain attribution to the author(s) and the title of the work, journal citation and DOI.
Introduction
Land surface phenology (LSP), which is the study of the seasonal dynamics of vegetated land surfaces from remote sensing, is a key indicator of global change, that both responds to and influences weather and climate (Richardson et al 2013, Rosenzweig et al 2007, Henebry and De Beurs 2013, De Beurs and Henebry 2004). Satellite imagery has been extensively used as a resource for the study of intra-annual vegetation dynamics at a variety of scales (White et al 2009, Primack and Miller-Rushing 2011), and to complement traditional plant phenological observations with information on the phenological status of vegetated land surfaces. This has been largely done through the analysis of time series of vegetation indices (VIs), which allow to quantify intra-annual changes in the timing and intensity of vegetation activity, and from which metrics such as the timing of the start, the end and the length of the growing season can be derived at various scales (Reed et al 2003).
The timing of the start and end, as well as the duration of the growing season, play a strong role in the seasonal atmosphere–biosphere exchange of energy, carbon and water (Richardson et al 2013). In turn, these seasonal changes are largely regulated by climatic conditions and in particular by three main factors: temperature, photoperiod and moisture availability (Jolly et al 2005). These climatic factors impose spatially and temporally variable constraints on vegetation activity (Körner and Basler 2010), which may be modelled at various scales (Stöckli et al 2008).
Previous studies have reported large-scale shifts in LSP metrics, namely start and end of season timing in particular over the Northern Hemisphere boreal and temperate zones (Julien and Sobrino 2009, Myneni et al 1997, Garonna et al 2016). Improving our understanding of which climatic factors influence the timing of leaf onset and senescence at large scales has been highlighted by previous studies as being crucial for estimating both climate change impacts on LSP and the potential feedbacks to climate (Mora et al 2015, Estiarte and Peñuelas 2015). Previous analyses derived the relative importance of climatic constraints on vegetation growth using mean climate (Nemani et al 2003) and from the intra-annual variation in vegetation activity (Jolly et al 2005). However, knowledge gaps on the relative importance of climatic constraints on LSP currently limit our ability to model LSP change at large scale and hence to predict changes in phenology under climate change (Richardson et al 2012, Delpierre et al 2016).
In this study, we examined three main climatic constraints on LSP at the start and end of the growing season at global scale and tested for temporal trends over the past three decades. We focused on three key climatic factors that drive phenological variability: minimum temperature, moisture availability (through vapour pressure deficit), and photoperiod (day length). Our aim was not only to test for trends, but also to examine whether the relative importance of these three constraints at the start and end of the growing season has shifted over the past decades. Given the LSP trends identified at global scale in previous studies (Garonna et al 2016, Buitenwerf et al 2015, Jeong et al 2011), we expected climatic constraints to have shifted over the past three decades, with repercussions on the relative importance of these constraints in many areas of the world and potentially reflecting shifting biotic communities (Saikkonen et al 2012).
Methods
Our approach involved disentangling the relative importance of three climatic constraint factors on vegetation growth at daily time steps, globally and for the period 1982–2011, using a modelled global phenology dataset (Stöckli et al 2011) in combination with the longest available global record of remotely-sensed leaf area index (LAI) observations (Zhu et al 2013). This methodology builds on the abundances derived from potential climatic constraints to plant growth from long-term climate statistics (Nemani et al 2003) as well as the intra-annual dynamics of these climatic constraints from previous studies (Jolly et al 2005). It is important to note that we consider in this study three climatic factors as constraints—not forcing factors—to LSP. Though meteorological factors may influence LSP both as forcing factors and as constraints, we consider here the day length, minimum daily temperature and daily evaporative demand as limiting resources. In other words, if any one of them is below a critical value at a given location at a particular time of the year it prevents the growth of vegetation.
Time series of leaf area index (1982–2011)
Two global LAI datasets—one modelled in response to climate and one remotely sensed—were used in combination for the period 1982–2011, which is the longest time period available for global VI records. Both LAI datasets are freely available through their respective references. The first is the Global Phenology Reanalysis (Stöckli et al 2011) and is referred to as LAIre in this paper. LAIre describes leaf development in response to climate and is thus chosen to represent 'climatically-induced' LAI. One advantage of this dataset is that, instead of simulating specific events of leaf development, it describes simulated temporal development of canopy greenness through LAI, which is directly comparable to the second dataset LAI3g. To produce these data, empirical parameters were used in combination with European Centre for Medium-Range Weather Forecast (ECMWF) ERA-Interim data in a prognostic mode. The empirical parameters were optimized using a data assimilation framework based on an Ensemble Kalman Filter with MODIS data, which was demonstrated as a useful approach to model phenology (Stöckli et al 2011).
The second LAI dataset is the LAI3g (Zhu et al 2013), which was used to represent remotely sensed observations of land surface phenology metrics at global scale for the past three decades. This dataset was developed with a neural network-based approach from the Normalized Difference Vegetation Index NDVI3g and Terra Moderate Resolution Imaging Spectroradiometer (MODIS) fraction of Photosynthetically Active Radiation (fAPAR) and LAI data products. Previous studies have used this dataset to derive both greening and browning trends and LSP trends (Xiao and Moody 2004, Liu et al 2010, Ni et al 2017, Cook and Pau 2013). LAI3g was thoroughly evaluated in Zhu et al (2013), who used comparisons with both ground-based LAI measurements and satellite products such as SPOT-Vegetation to demonstrate the quality and research applicability of this dataset for monitoring global vegetation dynamics.
As a first step, we compared LAI time series from both datasets, finding overall good agreement between them for most areas of the world (mean absolute error MAE = 0.32; and described in supplementary text 1 available at stacks.iop.org/ERL/13/024025/mmedia). In order to compare the two, we aggregated the LAI3g dataset from its native spatial resolution of 1/12 degrees to the LAIre 1/2-degree resolution, using the areal mean and omitting no-data pixels. Additionally, bi-monthly time steps of LAIre were extracted to coincide with the temporal resolution of LAI3g. For both datasets we considered only the overlapping record i.e. 1982–2011. Results from this step (as reported in the supplementary materials section) provided evidence that the modelled LAIre can reproduce within- and between-year LAI dynamics as observed in the remotely sensed dataset, and that the two datasets can be used in combination in the next steps of our analysis.
Three modelled climatic constraints to phenology
In producing the reanalysis data, Stöckli et al (2011) parameterized the light, moisture and temperature requirements of 35 Plant Functional Types (PFTs) by minimizing the cost function of globally predicted versus MODIS-observed LAI. The rationale behind this is that each PFT has an optimal set of climatic states suitable for photosynthesis. These empirical parameters are considered stable throughout the reanalysis timeframe. Then, ECMWF ERA-Interim data were used to re-analyse (by use of the prognostic LAI model combined with ensemble data assimilation of MODIS data) LAI on a daily basis for each grid cell, taking into account the fractional cover of PFTs within the cell (Stöckli et al 2011). The model predicting LAIre employs the growing season index (GSI), a bioclimatic index summarizing climatic constraints on leaf development (Jolly et al 2005). The daily GSI (iGSI) is calculated as the product of three indices of phenological response to time aggregated climatic states, as follows:

where iTMin, iVPD and iPhoto are the daily indices of phenological responses to time-aggregated minimum temperature (TMin), water vapour pressure deficit (VPD) and photoperiod (Photo) constraints, respectively (Jolly et al 2005). The climatic data were derived from ECMWF ERA-Interim daily minimum temperature, daily mean photoperiod and daily mean vapour pressure deficit data. Among these, daily mean photoperiod for a given location remains constant at decadal scale since it is determined as a function of latitude and time of year. The indices iTMin, iVPD and iPhoto were normalized to range between 0 and 1, with lower values signifying a greater limitation to leaf growth (Jolly et al 2005). Combined with structural parameters and embedded in a prognostic LAI state calculation, the Growing Season Index drives LAIre intra-annual variations.
From the GSI time series for each pixel we extracted a simple indicator of the daily constraint imposed by climate on leaf growth, simply defined as follows:

We also extracted the three climatic constraints on leaf development as defined in the reanalysis data. For each of the three GSI components, we derived the corresponding daily climatic constraint index (CC), which represents the degree to which each factor independently constrains vegetation activity during that day. The CC also ranges from 0 to 1, with 0 indicating no climatological constraint to photosynthesis and 1 representing climatic conditions that are completely constraining to vegetation activity. For instance, for VPD, the daily climatic constraint index CCVPD is calculated as CVPD = 1 − iVPD, where CCVPD is the daily constraint corresponding to iVPD. Figure 1 illustrates a yearly time series of iTmin, iVPD, iPhoto, iGSI and LAIre data for a grid cell in Siberia.
Figure 1. Reanalysis and climatic constraint data at a pixel in Siberia (65°N, 84°E) for 2009. DOY stands for day of year. The CC and GSI indices are presented in the top panel. The lower panel presents the corresponding LAIre and LAI3g observations for the same location, at 0.5 degree spatial resolution.
Download figure:
Standard image High-resolution imageExtraction of start and end of season metrics for 1982–2011
We derived start and end of season timing from remotely sensed LAI records (LAI3g) at their native spatial resolution of 1/12 degrees. We used LAI3g time series to extract LSP metrics because these data represent observed (rather than modelled) dynamics of the vegetated land surface. Start and end of season timing are both expressed in days of year (DOY). Based on previous studies (Garonna et al 2016, White et al 2009), we used harmonic noise filtering and extracted start and end of season metrics for each year using the commonly used Midpointpixel method, which defines start of the growing season as the first day of the year when LAI is greater than its midpoint during the year (i.e. half the annual range). Calendar years (January–December) were considered for Northern Hemisphere pixels, and July–June years were considered for Southern Hemisphere pixels.
Quantifying the three climatic constraints (CC) at start and end of season
For each pixel, we quantified climatic constraints at the start and end of season by summing the climatic constraint values over a pre-season of 21 days before the day of start (or end) of season for each given year, as derived from LAI3g. Instead of examining climatic constraints only on the very day of the start or the end of season we preferred using a 21 days window preceding these days to buffer against the effects of short-term meteorological events. We selected 21days as the pre-season duration based on the mean averaging time for VPD and Tmin used in developing the reanalysis (Stöckli et al 2011) as well as and given the smoothing-window length applied to the GSI (Jolly et al 2005). Although an optimal pre-season duration is likely to vary between biome and the climatic constraint considered (Shen et al 2014), we preferred one consistent approach for all situations (supplementary text 2), because this allowed us to compare the relative importance of the three constraints on LSP across environmental zones.
We examined trends in climatic constraints over the modelled LAIre over the study period for each pixel, in order to test whether they increased or decreased during the study period. Where trends were found, we considered the sign of the linear regression slope as indicative of decreasing/increasing climatic constraints over time. The magnitude of this slope factor was not considered as scientifically meaningful given the nature of the three indicators. We also calculated the Relative Importance (RI) of each climatic indicator to the overall constraint on start and end of season. More precisely, this means calculating the percentage of the total constraint over each pre-season (the 21 days preceding start or end of season day) that is due to each of the climatic factors. This is illustrated in equation (3) for VPD:
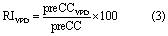
where RIVPD is the relative importance (in %) of CCVPD over the start of season pre-season (the 21 days preceding SOS), and preCCVPD and preCC are the CCVPD and total constraint over the pre-season (i.e. over the 21 days preceding either SOS or EOS). Furthermore, we defined the 'predominant' constraint over start or end of season for each year as the climatic factor with the highest total CC over the respective pre-season.
Environmental stratification and trend analysis
We stratified our results by environmental zone using the Global Environmental Stratification (GEnZ) dataset (Metzger et al 2013) aggregated at 0.5 degree spatial resolution. GEnZ classifies the global land area in environmental zones based on multi-variate clustering of bio-climatic data (Metzger et al 2013). Our study focused on the eight environmental zones for which our LSP extraction algorithm could obtain sufficiently reliable metrics. These were defined with a threshold of a minimum of 50% of the zone's area and a minimum of 500 pixels per zone. In the supplementary materials section, tables S1 and S2 detail the selection of the 8 global environmental zones based on these two criteria. Excluded global environmental zones include the artic/alpine and tropical biomes, the latter being also the biome with the weakest agreement between LAI3g and LAIre (supplementary text 1).
Figure 2. Geographic distribution of the eight global environmental zones considered in this study (left panel) and their respective annual average climatic constraint distribution over the period 1982–3011 (right panel). The global environmental zones are: ECM: extremely cold and mesic; CM: cold and mesic; CTD: cool temperate and dry; CTX: cool temperate and xeric; CTM: cool temperate and mesic; WTM: warm temperate and mesic; HD: hot and dry. The climatic constraints are: minimum temperature (TMin), photoperiod (Photo), vapour pressure deficit (VPD).
Download figure:
Standard image High-resolution imageThese 8 global environmental zones as well as their average climatic constraint distribution are presented in figure 2. They cover ~50% of all land area and encompass the boreal, temperate and dryland biomes. These correspond to those that were previously highlighted as hotspot areas of LSP change (Garonna et al 2016). In the Northern Hemisphere, the zones roughly correspond to latitudinal bands, ranging from the northernmost extremely cold and mesic (ECM) zone to the hot and dry (HD) zone. The latitudinal distribution is also reflected in their average climatic constraint distribution (figure 2, right panel).
Three types of pixels were excluded from our analyses, and their spatial distribution and extent are presented as supplementary materials (figure S1 and table S1). Firstly, given our focus on vegetated land areas, all pixels with over 50% water or bare coverage (bare soil, rock, ice and permanent snow) were omitted from our analysis. Fractional covers were derived from the PFT data used in the Global Reanalysis (Stöckli et al 2011). Secondly, pixels with over 50% cropland cover were also excluded because we consider the start and end of season timing of pixels with more than 50% cropland cover to be primarily influenced by human intervention rather than climatic conditions. Thirdly, pixels with no distinct seasonality or with low vegetation cover, which were flagged by the LSP extraction algorithm as presenting less reliable start and end of season estimates over the study period. The latter covered mostly tropical regions.
We detected significant trends in climatic constraints using both linear regression and a Mann-Kendall trend test, a non-parametric method to test for monotonic trends. The latter approach is known to be reliable and robust in cases of non-normality and missing values in time series (Mann 1945, De Beurs and Henebry 2005). Beside minor differences in the number of significant trends found, the overall results from Mann-Kendall trend and linear regression analyses were in agreement with one another. In mapping changes in climatic constraints, we only present positive or negative trends that were significant at the 5% confidence interval (p < 0.05) according to the Mann-Kendall trend test. When presenting average values over an environmental zone, we consider all pixels within that area aside from the three excluded types (predominantly bare or water-covered, croplands and without distinct seasonality). All area calculations were done after projecting data to the equal-area MODIS Sinusoidal projection.
Results
Figure 3 presents the climatic constraints on the start- and end-of-season dates for the eight global environmental zones considered, averaged over our study period (1982–2011). Firstly, for both start and end of season, multiple constraints appeared to act simultaneously. Secondly, we found considerable differences in the constraints acting at the start and end of the growing season for almost all zones considered. This is clearly visible in figure 3, where both the intensity and the mix of constraints differ significantly between start and end of season (top and bottom panels, respectively). At the start of season, TMin appeared to be largely constraining over most of the Northern Hemisphere, with some influence of photoperiod particularly over Western Europe and the Eastern United States. The area covered by VPD constraints was much larger at the end of season, including central and western North America, as well as southern Africa and central Eurasia. Overall, a different more and more complex mix of climatic constraints appeared to underlie the end of season.
Figure 3. Average regional climatic constraints (minimum temperature TMin, VPD and photoperiod) on satellite-derived start (top panel) and end (bottom panel) of the growing season, averaged over the study period (1982–2011). Excluded global environmental zones, as well as barren and water-covered pixels are shown in white.
Download figure:
Standard image High-resolution imageFigure 4. Decreasing and increasing trends in the total climatic constraint on the start or the end of the growing season during 1982–2011. Grey pixels belong to global environmental zones outside our study area. Pixels with colour correspond to areas displaying significant (p < 0.05) positive or negative trends in the CC index over the spring/autumn pre-season for 1982–2011.
Download figure:
Standard image High-resolution imageFigure 5. Average shift and standard error (in %) in the relative importance (RI) of three climatic constraints (TMin, VPD and Photo, respectively) on the start and the end of the growing season (left and right panels, respectively) for the 8 global environmental zones considered. The eight zones are: ECM: extremely cold and mesic; CM: cold and mesic; CTD: cool temperate and dry; CTX: cool temperate and xeric; CTM: cool temperate and mesic; WTM: warm temperate and mesic; HD: hot and dry. Horizontal bars represent percentage change in the relative importance of the three climatic constraints, averaged over each environmental zone for the period 1982–2011. The average number of pixels (in equal-area projection) with statistically significant change per environmental zone (N) is presented below each zone name. This number N is proportional the areal extent covered, as follows: area = N ⁎ 3087 km2. The average change in start and end of season timing (per zone) are presented in table S3 of the supplementary materials section.
Download figure:
Standard image High-resolution imageWe found significant (p < 0.05) trends in climatic constraints on the start and end of season (figure 4). These covered 18% of the 8 global environmental zones considered, and 10% of total land areas. Trends varied both spatially and temporally between start and end of season. At high northern latitudes (>50°N), we found negative trends in CC across the American and Eurasian continents (figure 4). In other words, at both start and end of the growing season, the overall constraint imposed by the three climatic factors decreased over the study period. Examining significant trends for each individual climatic constraint individually, we found the minimum temperature constraint to have eased over many areas of the northern high latitudes, particularly at end of season (figure S2). In the Southern Hemisphere, the temperature constraint on start of season increased in parts of central South America, Southern Africa and Australia (figure S2).
Increasing climatic constraints were found for both start and end of season over parts of western North America and Mexico and pocket regions such as Fennoscandia in Europe or in South America. These are mostly associated with both the photoperiod constraint, which appears to have increased at both start and end of the growing season in pocket areas of continental Europe and North America (figure S2), and the increase in the moisture constraint (represented by VPD). This was found at the start of season over Mexico and pocket areas of central Eurasia, and additionally at the end of season over eastern Pan-Europe and South-eastern Russia (figure S2). Overall, changes in the three constraints were larger for the end than for the start of the season and contrasting trends between start and end of season (with p < 0.05) covered only 1% of the study area.
We found that the relative importance of the three constraints on land surface phenology changed considerably during the study period, with average shifts in the relative importance of the three constraints reaching almost 8% over 1982–2011 for given environmental zones (figure 5). Despite considerable within-zone variation (in particular over the WTX zone), the relative importance of the temperature constraint on both start and end of season decreased on average across all zones, over a total area of 5.71 million km2 for start of season and 8.99 million km2 for end of season. The resulting average change in the relative importance of the moisture and photoperiod constraints varied among environmental zones and between start and end of season (figure 5).
For the start of season, the decreasing importance of the temperature constraint was counter-balanced by increasing photoperiod constraints across all boreal, temperate and dryland zones, except in cool temperate zones (CTD, CTX and CTM) where both moisture and photoperiod became more limiting. For the end of season, the decline in the temperature constraint was opposed mostly by an increase in the photoperiod constraint in the boreal biome and in the moisture constraint in the temperate (both cool and warm). On average, the moisture constraint increased considerably in its relative influence on both start and end of season of most environmental zones considered, independently of trends in LSP derived from LAI3g.
By examining only the constraint with the largest influence on start and end of season (referred to as 'predominant' constraint, figure S3), we found a significant increase of ~5% in the total area where VPD was the predominant constraint at end of season, during the study period. The total area where the temperature constraint was previously predominant decreased, and the relative importance of this constraint decreased in parallel with the growth in relative importance of the other two constraints.
Discussion
Understanding the relative importance of various meteorological factors at start and end of season in specific regions is important to shed light on the potential effects of climate change on land surface phenology (Estiarte and Peñuelas 2015). The notion that multiple constraints—rather than single limiting factors—determine phenological variation in most areas of the world has already been reported in previous literature for annual data (Churkina and Running 1998, Jolly et al 2005), but to our knowledge not for start and end of season. In a recent study, Madani et al (2017) use solar-induced chlorophyll fluorescence to find joint climatic constraint factors (in their study, VPD, TMin and soil moisture) on global ecosystem productivity. Our findings expand this point to specifically include spring and autumn processes. Our results also underline the importance of studying how climatic constraints vary in concert to influence large-scale phenological variability—as put forward by Forkel et al (2015). Although minimum temperature is in our results a dominant constraint during spring phenology over most of the Northern Hemisphere, figure 3 interestingly shows the importance of moisture and photoperiod constraints particularly at the end of season, as has already been put forward in previous studies (Liu et al 2016, Bauerle et al 2012).
Spring phenology has received more scientific attention as compared to senescence (Gallinat et al 2015). Our characterization of climatic constraints at the end of season—namely the weaker, more variable and more complex mix of climatic constraints as compared to start of season—is in line with the well-known difficulty to track autumn phenology in comparison to spring phenology (Gallinat et al 2015), and with the fact that autumn phenology is controlled by a larger suite of environmental cues (e.g. frost, wind) than spring phenology (Parmesan and Hanley 2015, Gill et al 2015).
Our results demonstrate the dynamic nature of climatic constraints on LSP. For both the start and the end of the growing season, we examined two types of shifts: trends in the total climatic constraint over the pre-season (figure 4) and in the relative importance of minimum temperature, moisture and photoperiod (figure 5). These changes in the importance of the three climatic constraints on global LSP may be due to either or both, climatic changes and LSP shifts over the time period from 1982–2011. This is especially evident when considering changes in the photoperiod constraint (figure S2 in supplementary materials): since the within-year variation in photoperiod does not vary between years for a given pixel (since it is determined by time-constant latitude in the global analysis), any trend in photoperiod constraint (as presented in figure S2, top panel) must be due to shifting LSP over the study period. Indeed, the areas presenting significant changes in the importance of the photoperiod constraint (e.g. across continental and boreal Europe or north-eastern America in figure S3) are also where LSP changes were identified using NDVI3g in another study (Garonna et al 2016). The fact that trends in climatic constraints were stronger for the end than for the start of the growing season is also consistent with the asymmetrically strong autumnal shifts found in previous studies over the same period (Jeong et al 2011).
Our analysis shows that, over the study period, minimum temperature became less constraining in all the environmental zones considered. The decrease in the minimum temperature constraint over the Northern Hemisphere high latitudes (figure 4 and S2) is consistent with the overall 'easing' of temperate conditions for plant growth in these areas over the last decades (Wang et al 2011, Myneni et al 1997, Zhou et al 2001, Nemani et al 2003, Lucht et al 2002). This 'relaxing' of the minimum temperature constraint is visible also through the diminishing relative importance of TMin as a constraint in all considered environmental zones (figure 5). This decrease was strongest in boreal zones (ECM and CM) in figure 5.
For the start of season, the decrease in the relative importance of minimum temperature as a constraint is met with an increase in the relative importance of photoperiod increases in all environmental zones considered (figure 5). In other words, regional warming in these areas allowed vegetation to meet its minimum temperature requirements earlier in the year, thus 'relaxing' the minimum temperature constraint over these zones and shifting the start of season to earlier in the year. Given that many plant species have evolved day-length requirements to match their phenology to the expected temperature pattern (Körner and Basler 2010, Basler and Körner 2012), the resulting shorter day length at the start of season points towards an increasing photoperiod constraint of the start of season in the eight global environmental zones considered. This assumes the day-length requirements of plants as stable, which appears reasonable in a 30 year timespan. Nevertheless, it is important to state that, firstly, the minimum requirement thresholds (assumed stable in the reanalysis) may also shift because of changing species distribution and evolution, which are likely to play a role in longer timescales. Secondly, our study does not consider cloudiness, which is a determinant of both light quantity and quality variations during the year.
For the end of season, where average shifts in constraints are greater, we found a widespread increase in the influence of moisture at the expense of a reduced influence of minimum temperature (figure 5 and S3). This adds to the importance of moisture as a constraint to land surface phenology at large scales, which has been demonstrated particularly for start of season and peak greenness over the past 30 years (Forkel et al 2015). Furthermore, our results show that this increasing importance of moisture has repercussions on constraint predominance for the end of season i.e. an increase in predominantly moisture limited areas over the study period (figure S3). Finally, it should be noted that our 50% threshold for excluding croplands is unlikely to exclude the effects of crop phenology in the detected LSP entirely (Zhang et al 2017). Moreover, our analysis did not take into account land-use/land-cover changes and fire, which may have large annual effects on land surface phenology (Forkel et al 2015). In areas where land management is the strongest driver of land surface phenology, our focus on climatic factors only is a limitation. This is visible in our results for example over the boreal and continental areas of Europe, which have been shown to have undergone growing season lengthening over the last decades (Garonna et al 2014) and which presented increasing climatic constraints on either start or end of season in our results (figure 4). In these areas, land management and use/land cover change is known to be a strong driver of land surface phenology change (Karlsen et al 2009, Fuchs et al 2013, Park et al 2015). Another limitation of our analysis is that it does not consider potential effects of increased or decreased nutrient availability or the CO2 fertilization increase within our study period (Schimel et al 2015, McLauchlan et al 2017), as well as potential changes in minimum requirements for growth within each plant functional type or changes in incoming radiation on vegetated land surfaces (which could be indicated by changing cloud cover, for example).
Conclusion
On top of demonstrating the value of reanalysis data to investigate the influence of specific climatic constraints to land surface phenology at large spatial and temporal scales, our study reveals shifts in the relative importance of climatic constraints in the temperate and boreal biomes over the study period. As temperature constraints appeared to ease widely across the environmental zones considered, a main finding of our study is the considerable increase in the relative influence of the moisture constraint both at the start and end of the growing season (over 5.71 million km2 and 8.99 million km2, respectively).
Acknowledgments
We thank R Myneni and colleagues for providing the LAI3g dataset, as well as J Pinzon and C J Tucker for providing the NDVI3g dataset. We are very grateful to the Functional Genomics Center Zurich for facilitating our data analysis and providing technical support, in particular to M Hakateyama, H Rehrauer and K Shimizu. This study has been conducted with the support of the University of Zurich Research Priority Program on Global Change and Biodiversity (URPP GCB). We are very grateful to the three anonymous reviewers for their valuable comments and feedback, which substantially improved this manuscript.