-
Asymptotics of the Least Trimmed Squares Estimator
-
A Causal Evaluation of the UK’s Mini-Budget Policy on Stock Market
-
Elimination Algorithms for Skew Polynomials with Applications in Cybersecurity
-
A Projection-Type Implicit Algorithm for Finding a Common Solution for Fixed Point Problems and Variational Inequality Problems
Journal Description
Mathematics
Mathematics
is a peer-reviewed, open access journal which provides an advanced forum for studies related to mathematics, and is published semimonthly online by MDPI. The European Society for Fuzzy Logic and Technology (EUSFLAT) and International Society for the Study of Information (IS4SI) are affiliated with Mathematics and their members receive a discount on article processing charges.
- Open Access— free for readers, with article processing charges (APC) paid by authors or their institutions.
- High Visibility: indexed within Scopus, SCIE (Web of Science), RePEc, and other databases.
- Journal Rank: JCR - Q1 (Mathematics) / CiteScore - Q1 (General Mathematics )
- Rapid Publication: manuscripts are peer-reviewed and a first decision is provided to authors approximately 17.1 days after submission; acceptance to publication is undertaken in 2.6 days (median values for papers published in this journal in the first half of 2024).
- Recognition of Reviewers: reviewers who provide timely, thorough peer-review reports receive vouchers entitling them to a discount on the APC of their next publication in any MDPI journal, in appreciation of the work done.
- Sections: published in 13 topical sections.
- Companion journals for Mathematics include: Foundations, Analytics, International Journal of Topology, Geometry and Logics.
Impact Factor:
2.3 (2023);
5-Year Impact Factor:
2.2 (2023)
Latest Articles
Recursive Estimation of the Expectile-Based Shortfall in Functional Ergodic Time Series
Mathematics 2024, 12(24), 3956; https://doi.org/10.3390/math12243956 - 16 Dec 2024
Abstract
This paper considers the Recursive Kernel Estimator (RKE) of the expectile-based conditional shortfall. The estimator is constructed under a functional structure based on the ergodicity assumption. More preciously, we assume that the input-variable is valued in a pseudo-metric space, output-variable is scalar and
[...] Read more.
This paper considers the Recursive Kernel Estimator (RKE) of the expectile-based conditional shortfall. The estimator is constructed under a functional structure based on the ergodicity assumption. More preciously, we assume that the input-variable is valued in a pseudo-metric space, output-variable is scalar and both are sampled from ergodic functional time series data. We establish the complete convergence rate of the RKE-estimator of the considered functional shortfall model using standard assumptions. We point out that the ergodicity assumption constitutes a relevant alternative structure to the mixing time series dependency. Thus, the results of this paper allows to cover a large class of functional time series for which the mixing assumption is failed to check. Moreover, the obtained results is established in a general way, allowing to particularize this convergence rate for many special situations including the kernel method, the independence case and the multivariate case. Finally, a simulation study is carried out to illustrate the finite sample performance of the RKE-estimator. In order to examine the feasibility of the recursive estimator in practice we consider a real data example based on financial time series data.
Full article
(This article belongs to the Special Issue Advances in High-Dimensional Statistics)
Open AccessArticle
Applying Theorems on b-Metric Spaces to Differential and Integral Equations Through Connected-Image Contractions
by
Khuanchanok Chaichana, Kanyuta Poochinapan, Teeranush Suebcharoen and Phakdi Charoensawan
Mathematics 2024, 12(24), 3955; https://doi.org/10.3390/math12243955 - 16 Dec 2024
Abstract
This paper introduces a new concept of a connected-image set for a mapping, which extends the notion of edge-preserving properties with respect to mapping. We also present novel definitions of connected-image contractions, with a focus on fixed-point theorems involving auxiliary functions in b
[...] Read more.
This paper introduces a new concept of a connected-image set for a mapping, which extends the notion of edge-preserving properties with respect to mapping. We also present novel definitions of connected-image contractions, with a focus on fixed-point theorems involving auxiliary functions in b-metric spaces. The relationships between these mathematical concepts are explored, along with their applications to solving differential and integral equations. In particular, we discuss existence results for solving integral equations and second-order ordinary differential equations with inhomogeneous Dirichlet boundary conditions, as well as theorems related to contractions of the integral type.
Full article
(This article belongs to the Special Issue Fixed Point, Optimization, and Applications II)
Open AccessArticle
Numerical Study of the Thermal Energy Storage Container Shape Impact on the NePCM Melting Process
by
Obai Younis, Naef A. A. Qasem, Aissa Abderrahmane and Abdeldjalil Belazreg
Mathematics 2024, 12(24), 3954; https://doi.org/10.3390/math12243954 - 16 Dec 2024
Abstract
Recently, thermal energy storage has emerged as one of the alternative solutions to increase energy efficiency. The geometry of a thermal energy storage container holds a significant role in increasing the heat transmission rates in the container. In this article, we examined the
[...] Read more.
Recently, thermal energy storage has emerged as one of the alternative solutions to increase energy efficiency. The geometry of a thermal energy storage container holds a significant role in increasing the heat transmission rates in the container. In this article, we examined the influence of the inner and outer tube shapes of a shell and tube LHTES on the thermal activity within the system. The gap between the inner and outer tube is loaded with nano-enhanced phase change material (NePCM); hot fluid is passed through the inner tube while the outer tube is insulated. COMSOL commercial software was used to numerically simulate the NePCM melting process. Mainly, six different geometries were investigated with inner or outer tubes with trefoil, cinquefoil, and heptafoil shapes. The influences of nanoparticles volumetric fraction (ϕ = 0–8%) were also discussed. The findings are displayed and discussed in terms of the average Nusselt number, the average liquid fraction, the total energy generation, and the average temperature. The findings showed that the melting process is highly affected by the shape of the inner tube and ϕ, while the outer tube shape impact is less important. It was noticed that employing an inner tube with a trefoil improved the melting process by more than 25% while increasing the ϕ from 0 to 8% resulted in reducing the melting time by up to 20%.
Full article
(This article belongs to the Special Issue Numerical Solution of Differential Equations and Their Applications)
Open AccessArticle
On a Numerical Solution to an Inverse Structural Density Problem with a Method of Local Corrections
by
Alexander Tsidaev and Igor Ladovskii
Mathematics 2024, 12(24), 3953; https://doi.org/10.3390/math12243953 - 16 Dec 2024
Abstract
The structural gravimetry problem, which involves determining the geometry of a contact surface between two homogeneous layers based on observed gravity fields, is addressed in this paper. The method of local corrections is presented in a generalized form to improve its applicability to
[...] Read more.
The structural gravimetry problem, which involves determining the geometry of a contact surface between two homogeneous layers based on observed gravity fields, is addressed in this paper. The method of local corrections is presented in a generalized form to improve its applicability to a broader range of problems. This study introduces several improvements to the local corrections method, including the use of a finite element approach for more accurate field calculations, particularly for near-surface boundaries. Additionally, the method incorporates prior knowledge of the boundary geometry, which serves as an initial approximation to enhance convergence and avoid potential divergence issues. Demonstrations on several synthetic examples are performed, which show the advantages of the generalized form of the method. For the territory of the Middle Urals, Russia, the refinement of two crustal boundaries is performed (the Moho boundary and middle crust boundary).
Full article
(This article belongs to the Section Computational and Applied Mathematics)
Open AccessFeature PaperArticle
On (i)-Curves in Blowups of
by
Olivia Dumitrescu and Rick Miranda
Mathematics 2024, 12(24), 3952; https://doi.org/10.3390/math12243952 - 16 Dec 2024
Abstract
In this paper, we study -curves with in the blown-up projective space in general points. The notion of -curves was analyzed in the early
[...] Read more.
In this paper, we study -curves with in the blown-up projective space in general points. The notion of -curves was analyzed in the early days of mirror symmetry by Kontsevich, with the motivation of counting curves on a Calabi–Yau threefold. In dimension two, Nagata studied planar -curves in order to construct a counterexample to Hilbert’s 14th problem. We introduce the notion of classes of - and -curves in with s points blown up, and we prove that their number is finite if and only if the space is a Mori Dream Space. We further introduce a bilinear form on a space of curves and a unique symmetric Weyl-invariant class, F (which we will refer to as the anticanonical curve class). For Mori Dream Spaces, we prove that -curves can be defined arithmetically by the linear and quadratic invariants determined by the bilinear form. Moreover, - and -Weyl lines give the extremal rays for the cone of movable curves in with points blown up. As an application, we use the technique of movable curves to reprove that if then Y is not a Mori Dream Space, and we propose to apply this technique to other spaces.
Full article
(This article belongs to the Special Issue Advanced Algebraic Geometry and Applications)
Open AccessArticle
Applications of Disaffinity Vectors to Certain Riemannian Manifolds
by
Hanan Alohali, Sharief Deshmukh and Bang-Yen Chen
Mathematics 2024, 12(24), 3951; https://doi.org/10.3390/math12243951 - 16 Dec 2024
Abstract
A disaffinity vector on a Riemannian manifold is a vector field whose affinity tensor vanishes. In this paper, we prove that every disaffinity vector on a compact Riemannian manifold is an incompressible vector field. Then, we discover a sufficient condition for an incompressible
[...] Read more.
A disaffinity vector on a Riemannian manifold is a vector field whose affinity tensor vanishes. In this paper, we prove that every disaffinity vector on a compact Riemannian manifold is an incompressible vector field. Then, we discover a sufficient condition for an incompressible vector field to be disaffinity. Next, we study trans-Sasakian 3-manifolds whose Reeb vector field is disaffinity and obtain two sufficient conditions for a trans-Sasakian 3-manifold to be homothetic to a Sasakian 3-manifold. Finally, we prove that a complete Riemannian manifold admitting a non-harmonic disaffinity function satisfying the Eikonal equation and a Ricci curvature inequality is isometric to a Euclidean space.
Full article
(This article belongs to the Special Issue Recent Studies in Differential Geometry and Its Applications)
Open AccessArticle
DeepONet-Inspired Architecture for Efficient Financial Time Series Prediction
by
Zeeshan Ahmad, Shudi Bao and Meng Chen
Mathematics 2024, 12(24), 3950; https://doi.org/10.3390/math12243950 - 16 Dec 2024
Abstract
Financial time series prediction is a fundamental problem in investment and risk management. Deep learning models, such as multilayer perceptrons, Convolutional Neural Networks (CNNs), and Long Short-Term Memory (LSTM), have been widely used in modeling time series data by incorporating historical information. Among
[...] Read more.
Financial time series prediction is a fundamental problem in investment and risk management. Deep learning models, such as multilayer perceptrons, Convolutional Neural Networks (CNNs), and Long Short-Term Memory (LSTM), have been widely used in modeling time series data by incorporating historical information. Among them, LSTM has shown excellent performance in capturing long-term temporal dependencies in time-series data, owing to its enhanced internal memory mechanism. In spite of the success of these models, it is observed that in the presence of sharp changing points, these models fail to perform. To address this problem, we propose, in this article, an innovative financial time series prediction method inspired by the Deep Operator Network (DeepONet) architecture, which uses a combination of transformer architecture and a one-dimensional CNN network for processing feature-based information, followed by an LSTM based network for processing temporal information. It is therefore named the CNN–LSTM–Transformer (CLT) model. It not only incorporates external information to identify latent patterns within the financial data but also excels in capturing their temporal dynamics. The CLT model adapts to evolving market conditions by leveraging diverse deep-learning techniques. This dynamic adaptation of the CLT model plays a pivotal role in navigating abrupt changes in the financial markets. Furthermore, the CLT model improves the long-term prediction accuracy and stability compared with state-of-the-art existing deep learning models and also mitigates adverse effects of market volatility. The experimental results show the feasibility and superiority of the proposed CLT model in terms of prediction accuracy and robustness as compared to existing prediction models. Moreover, we posit that the innovation encapsulated in the proposed DeepONet-inspired CLT model also holds promise for applications beyond the confines of finance, such as remote sensing, data mining, natural language processing, and so on.
Full article
(This article belongs to the Section Financial Mathematics)
►▼
Show Figures
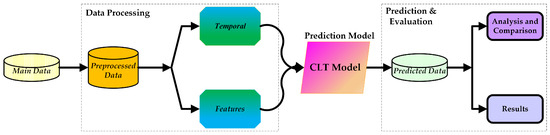
Figure 1
Figure 1
<p>Analysis flowchart of the stock price prediction.</p> Full article ">Figure 2
<p>Schematic diagram of the DeepONet.</p> Full article ">Figure 3
<p>Proposed DeepONet-inspired architecture of the CLT model.</p> Full article ">Figure 4
<p>Data sampling method.</p> Full article ">Figure 5
<p>Loss curve on training and validation sets.</p> Full article ">Figure 6
<p>Comparison of the prediction accuracy with varying number of nodes in a hidden layer.</p> Full article ">Figure 7
<p>Comparison of the prediction accuracy with varying number of hidden layers.</p> Full article ">Figure 8
<p>Comparison of the results produced by various models for the AEX.</p> Full article ">Figure 9
<p>Comparison of the results produced by various models for the ATX.</p> Full article ">Figure 10
<p>Model performance variation.</p> Full article ">Figure A1
<p>Comparison of the results produced by various models for the following: (<b>a</b>) FCHI; (<b>b</b>) FTSE; (<b>c</b>) HSI; (<b>d</b>) JKSE; (<b>e</b>) KLSE; (<b>f</b>) OEX.</p> Full article ">
<p>Analysis flowchart of the stock price prediction.</p> Full article ">Figure 2
<p>Schematic diagram of the DeepONet.</p> Full article ">Figure 3
<p>Proposed DeepONet-inspired architecture of the CLT model.</p> Full article ">Figure 4
<p>Data sampling method.</p> Full article ">Figure 5
<p>Loss curve on training and validation sets.</p> Full article ">Figure 6
<p>Comparison of the prediction accuracy with varying number of nodes in a hidden layer.</p> Full article ">Figure 7
<p>Comparison of the prediction accuracy with varying number of hidden layers.</p> Full article ">Figure 8
<p>Comparison of the results produced by various models for the AEX.</p> Full article ">Figure 9
<p>Comparison of the results produced by various models for the ATX.</p> Full article ">Figure 10
<p>Model performance variation.</p> Full article ">Figure A1
<p>Comparison of the results produced by various models for the following: (<b>a</b>) FCHI; (<b>b</b>) FTSE; (<b>c</b>) HSI; (<b>d</b>) JKSE; (<b>e</b>) KLSE; (<b>f</b>) OEX.</p> Full article ">
Open AccessEditorial
Advances in Fuzzy Logic and Artificial Neural Networks
by
Francisco Rodrigues Lima-Junior
Mathematics 2024, 12(24), 3949; https://doi.org/10.3390/math12243949 - 16 Dec 2024
Abstract
Fuzzy logic and artificial neural networks are among the most prominent AI approaches, recognized for their importance across various domains [...]
Full article
(This article belongs to the Special Issue Advances in Fuzzy Logic and Artificial Neural Networks)
►▼
Show Figures
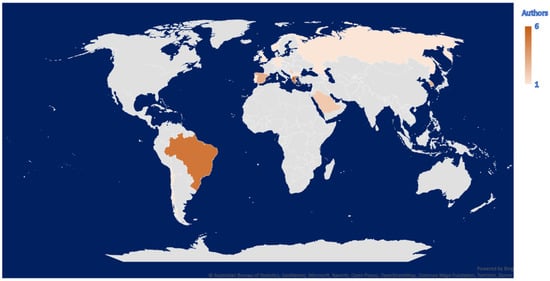
Figure 1
Open AccessArticle
A Dynamic Hill Cipher with Arnold Scrambling Technique for Medical Images Encryption
by
Yuzhou Xi, Yu Ning, Jie Jin and Fei Yu
Mathematics 2024, 12(24), 3948; https://doi.org/10.3390/math12243948 - 15 Dec 2024
Abstract
Cryptography is one of the most important branches of information security. Cryptography ensures secure communication and data privacy, and it has been increasingly applied in healthcare and related areas. As a significant cryptographic method, the Hill cipher has attracted significant attention from experts
[...] Read more.
Cryptography is one of the most important branches of information security. Cryptography ensures secure communication and data privacy, and it has been increasingly applied in healthcare and related areas. As a significant cryptographic method, the Hill cipher has attracted significant attention from experts and scholars. To enhance the security of the traditional Hill cipher (THC) and expand its application in medical image encryption, a novel dynamic Hill cipher with Arnold scrambling technique (DHCAST) is proposed in this work. Unlike the THC, the proposed DHCAST uses a time-varying matrix as its secret key, which greatly increases the security of the THC, and the new DHCAST is successfully applied in medical images encryption. In addition, the new DHCAST method employs the Zeroing Neural Network (ZNN) in its decryption to find the time-varying inversion key matrix (TVIKM). In order to enhance the efficiency of the ZNN for solving the TVIKM, a new fuzzy zeroing neural network (NFZNN) model is constructed, and the convergence and robustness of the NFZNN model are validated by both theoretical analysis and experiment results. Simulation experiments show that the convergence time of the NFZNN model is about 0.05 s, while the convergence time of the traditional Zeroing Neural Network (TZNN) model is about 2 s, which means that the convergence speed of the NFZNN model is about 400 times that of the TZNN model. Moreover, the Peak Signal to Noise Ratio (PSNR) and Number of Pixel Change Rate (NPCR) of the proposed DHCAST algorithm reach 9.51 and 99.74%, respectively, which effectively validates its excellent encryption quality and attack prevention ability.
Full article
(This article belongs to the Special Issue Chaotic Systems and Their Applications, 2nd Edition)
►▼
Show Figures
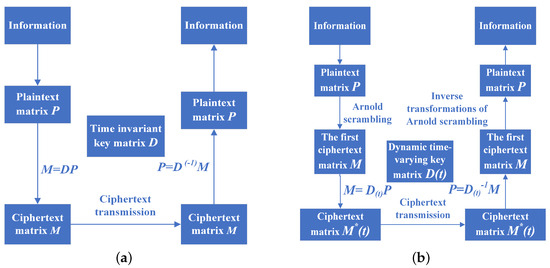
Figure 1
Figure 1
<p>The THC and proposed DHCAST. (<b>a</b>) The THC. (<b>b</b>) The proposed DHCAST.</p> Full article ">Figure 2
<p>The structure of FLS.</p> Full article ">Figure 3
<p>State solutions and residual errors of the NFZNN and other models for solving the TVIKM <math display="inline"><semantics> <mrow> <mi>A</mi> <mrow> <mo>(</mo> <mi>t</mi> <mo>)</mo> </mrow> <mo>=</mo> <msup> <mi>D</mi> <mrow> <mo>−</mo> <mn>1</mn> </mrow> </msup> <mrow> <mo>(</mo> <mi>t</mi> <mo>)</mo> </mrow> </mrow> </semantics></math> without noise. (<b>a</b>) State solutions of the models without noise. (<b>b</b>) Residual errors of the models without noise.</p> Full article ">Figure 4
<p>State solutions and residual errors of the NFZNN model and other models for solving the TVIKM matrix <math display="inline"><semantics> <mrow> <mi>A</mi> <mrow> <mo>(</mo> <mi>t</mi> <mo>)</mo> </mrow> <mo>=</mo> <msup> <mi>D</mi> <mrow> <mo>−</mo> <mn>1</mn> </mrow> </msup> <mrow> <mo>(</mo> <mi>t</mi> <mo>)</mo> </mrow> </mrow> </semantics></math> with combined noises. (<b>a</b>) State solutions of the models with combined noises. (<b>b</b>) Residual errors of the models with combined noises.</p> Full article ">Figure 5
<p>The DHCAST encryption and decryption simulation results for an ultrasound cardiology color image <math display="inline"><semantics> <mrow> <mo>(</mo> <mn>528</mn> <mo>×</mo> <mn>528</mn> <mo>×</mo> <mn>3</mn> <mo>)</mo> </mrow> </semantics></math>. (<b>a</b>) Key time <math display="inline"><semantics> <mrow> <mi>t</mi> <mo>=</mo> <mn>5.7</mn> </mrow> </semantics></math> s. (<b>b</b>) Key time <math display="inline"><semantics> <mrow> <mi>t</mi> <mo>=</mo> <mn>14.3</mn> </mrow> </semantics></math> s.</p> Full article ">Figure 6
<p>The DHCAST encryption and decryption simulation results of MRI grayscale image <math display="inline"><semantics> <mrow> <mo>(</mo> <mn>528</mn> <mo>×</mo> <mn>528</mn> <mo>×</mo> <mn>1</mn> <mo>)</mo> </mrow> </semantics></math>. (<b>a</b>) Key time <math display="inline"><semantics> <mrow> <mi>t</mi> <mo>=</mo> <mn>5.7</mn> </mrow> </semantics></math> s. (<b>b</b>) Key time <math display="inline"><semantics> <mrow> <mi>t</mi> <mo>=</mo> <mn>14.3</mn> </mrow> </semantics></math> s.</p> Full article ">Figure 6 Cont.
<p>The DHCAST encryption and decryption simulation results of MRI grayscale image <math display="inline"><semantics> <mrow> <mo>(</mo> <mn>528</mn> <mo>×</mo> <mn>528</mn> <mo>×</mo> <mn>1</mn> <mo>)</mo> </mrow> </semantics></math>. (<b>a</b>) Key time <math display="inline"><semantics> <mrow> <mi>t</mi> <mo>=</mo> <mn>5.7</mn> </mrow> </semantics></math> s. (<b>b</b>) Key time <math display="inline"><semantics> <mrow> <mi>t</mi> <mo>=</mo> <mn>14.3</mn> </mrow> </semantics></math> s.</p> Full article ">
<p>The THC and proposed DHCAST. (<b>a</b>) The THC. (<b>b</b>) The proposed DHCAST.</p> Full article ">Figure 2
<p>The structure of FLS.</p> Full article ">Figure 3
<p>State solutions and residual errors of the NFZNN and other models for solving the TVIKM <math display="inline"><semantics> <mrow> <mi>A</mi> <mrow> <mo>(</mo> <mi>t</mi> <mo>)</mo> </mrow> <mo>=</mo> <msup> <mi>D</mi> <mrow> <mo>−</mo> <mn>1</mn> </mrow> </msup> <mrow> <mo>(</mo> <mi>t</mi> <mo>)</mo> </mrow> </mrow> </semantics></math> without noise. (<b>a</b>) State solutions of the models without noise. (<b>b</b>) Residual errors of the models without noise.</p> Full article ">Figure 4
<p>State solutions and residual errors of the NFZNN model and other models for solving the TVIKM matrix <math display="inline"><semantics> <mrow> <mi>A</mi> <mrow> <mo>(</mo> <mi>t</mi> <mo>)</mo> </mrow> <mo>=</mo> <msup> <mi>D</mi> <mrow> <mo>−</mo> <mn>1</mn> </mrow> </msup> <mrow> <mo>(</mo> <mi>t</mi> <mo>)</mo> </mrow> </mrow> </semantics></math> with combined noises. (<b>a</b>) State solutions of the models with combined noises. (<b>b</b>) Residual errors of the models with combined noises.</p> Full article ">Figure 5
<p>The DHCAST encryption and decryption simulation results for an ultrasound cardiology color image <math display="inline"><semantics> <mrow> <mo>(</mo> <mn>528</mn> <mo>×</mo> <mn>528</mn> <mo>×</mo> <mn>3</mn> <mo>)</mo> </mrow> </semantics></math>. (<b>a</b>) Key time <math display="inline"><semantics> <mrow> <mi>t</mi> <mo>=</mo> <mn>5.7</mn> </mrow> </semantics></math> s. (<b>b</b>) Key time <math display="inline"><semantics> <mrow> <mi>t</mi> <mo>=</mo> <mn>14.3</mn> </mrow> </semantics></math> s.</p> Full article ">Figure 6
<p>The DHCAST encryption and decryption simulation results of MRI grayscale image <math display="inline"><semantics> <mrow> <mo>(</mo> <mn>528</mn> <mo>×</mo> <mn>528</mn> <mo>×</mo> <mn>1</mn> <mo>)</mo> </mrow> </semantics></math>. (<b>a</b>) Key time <math display="inline"><semantics> <mrow> <mi>t</mi> <mo>=</mo> <mn>5.7</mn> </mrow> </semantics></math> s. (<b>b</b>) Key time <math display="inline"><semantics> <mrow> <mi>t</mi> <mo>=</mo> <mn>14.3</mn> </mrow> </semantics></math> s.</p> Full article ">Figure 6 Cont.
<p>The DHCAST encryption and decryption simulation results of MRI grayscale image <math display="inline"><semantics> <mrow> <mo>(</mo> <mn>528</mn> <mo>×</mo> <mn>528</mn> <mo>×</mo> <mn>1</mn> <mo>)</mo> </mrow> </semantics></math>. (<b>a</b>) Key time <math display="inline"><semantics> <mrow> <mi>t</mi> <mo>=</mo> <mn>5.7</mn> </mrow> </semantics></math> s. (<b>b</b>) Key time <math display="inline"><semantics> <mrow> <mi>t</mi> <mo>=</mo> <mn>14.3</mn> </mrow> </semantics></math> s.</p> Full article ">
Open AccessArticle
Module Configuration of Rail Freight Transportation with Both Customer Segmentation and Product Family Genealogy in China
by
Weiya Chen, Shiying Tong, Ziyue Yuan and Xiaoping Fang
Mathematics 2024, 12(24), 3947; https://doi.org/10.3390/math12243947 - 15 Dec 2024
Abstract
The Chinese government is actively restructuring transportation to shift towards more sustainable rail freight transportation (RFT); however, there is still a lack of more systematic optimization in the whole production chain. This study develops a dual-focus modular configuration approach to explore the integration
[...] Read more.
The Chinese government is actively restructuring transportation to shift towards more sustainable rail freight transportation (RFT); however, there is still a lack of more systematic optimization in the whole production chain. This study develops a dual-focus modular configuration approach to explore the integration of customer demand and the production chain to achieve more sustainable operations in RFT. Customers have yielded eleven distinct groups, and operational processes have been segmented into sixteen modules by using the Ant Colony Optimization-based Fuzzy C-Means Clustering (ACOFCM) algorithm. Consequently, a Product Family Genealogy (PFG) model is conducted to identify three tailored product families (i.e., cross-border, multi-modal and general freight product). The developed dual-focus modular configuration approach has been proven to be feasible by utilizing a backtracking algorithm through a case study in an RFT logistics enterprise in China, which provides a standardization and optimization for RFT modular configurations.
Full article
(This article belongs to the Special Issue Optimization in Sustainable Transport and Logistics)
►▼
Show Figures
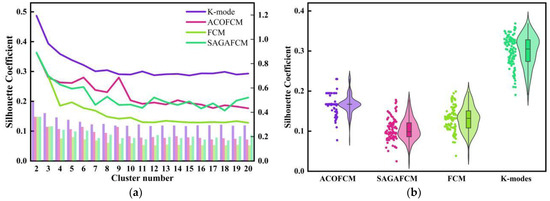
Figure 1
Figure 1
<p>Comparison of different cluster numbers. (<b>a</b>) Silhouette Coefficients of different cluster numbers. (<b>b</b>) Comparison of external indicator iterations.</p> Full article ">Figure 2
<p>Correlation coefficient of customer demands.</p> Full article ">Figure 3
<p>The modular division results of RFT product.</p> Full article ">Figure 4
<p>Demand and functional attributes of each modules. (<b>a</b>) Demand attributes of each modules. (<b>b</b>) Functional attributes of each modules.</p> Full article ">Figure 5
<p>The whole scheme of product family group, modules and customer groups.</p> Full article ">Figure 6
<p>The framework for product module configuration model building.</p> Full article ">
<p>Comparison of different cluster numbers. (<b>a</b>) Silhouette Coefficients of different cluster numbers. (<b>b</b>) Comparison of external indicator iterations.</p> Full article ">Figure 2
<p>Correlation coefficient of customer demands.</p> Full article ">Figure 3
<p>The modular division results of RFT product.</p> Full article ">Figure 4
<p>Demand and functional attributes of each modules. (<b>a</b>) Demand attributes of each modules. (<b>b</b>) Functional attributes of each modules.</p> Full article ">Figure 5
<p>The whole scheme of product family group, modules and customer groups.</p> Full article ">Figure 6
<p>The framework for product module configuration model building.</p> Full article ">
Open AccessArticle
Analytical Shortcuts to Multiple-Objective Portfolio Optimization: Investigating the Non-Negativeness of Portfolio Weight Vectors of Equality-Constraint-Only Models and Implications for Capital Asset Pricing Models
by
Yue Qi, Yue Wang, Jianing Huang and Yushu Zhang
Mathematics 2024, 12(24), 3946; https://doi.org/10.3390/math12243946 - 15 Dec 2024
Abstract
Computing optimal-solution sets has long been a topic in multiple-objective optimization. Despite substantial progress, there are still research limitations in the multiple-objective portfolio optimization area. The optimal-solution sets’ structure is barely known. Public-domain software for even three objectives is absent. Alternatively, researchers scrutinize
[...] Read more.
Computing optimal-solution sets has long been a topic in multiple-objective optimization. Despite substantial progress, there are still research limitations in the multiple-objective portfolio optimization area. The optimal-solution sets’ structure is barely known. Public-domain software for even three objectives is absent. Alternatively, researchers scrutinize equality-constraint-only models and analytically resolve them. Within this context, this paper extends these analytical methods for nonnegative constraints and thus theoretically contributes to the literature. We prove the existence of positive elements and negative elements for the optimal-solution sets. Practically, we prove that non-negative subsets of the optimal-solution sets can exist. Consequently, the possible existence endorses these analytical methods, because researchers bypass mathematical programming, analytically resolve, and pinpoint some non-negative optima. Moreover, we elucidate these analytical methods’ alignment with capital asset pricing models (CAPMs). Furthermore, we generalize for k-objective models. In conclusion, this paper theoretically reinforces these analytical methods and hints the optimal-solution sets’ structure for multiple-objective portfolio optimization.
Full article
(This article belongs to the Special Issue Mathematical Models and Applications in Finance)
►▼
Show Figures
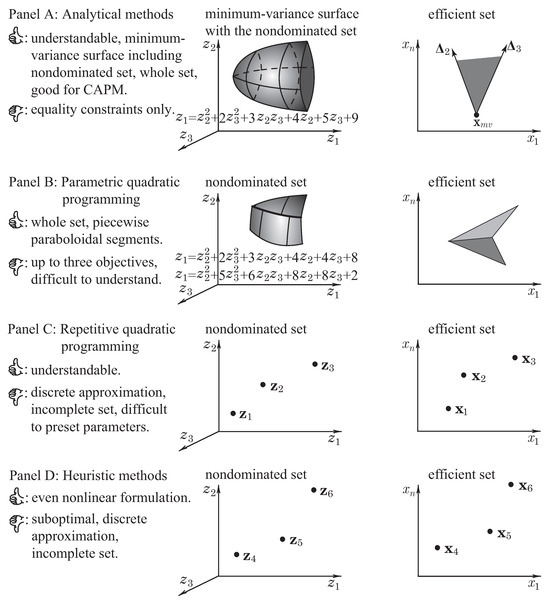
Figure 1
Figure 1
<p>Comparing methods to resolve (<a href="#FD4-mathematics-12-03946" class="html-disp-formula">4</a>) with the criterion space in the left part and decision space respectively in the right part.</p> Full article ">Figure 2
<p>A flow chart of searching a non-negative subset.</p> Full article ">Figure 3
<p>A convex set and efficient sets of (<a href="#FD3-mathematics-12-03946" class="html-disp-formula">3</a>), (<a href="#FD2-mathematics-12-03946" class="html-disp-formula">2</a>), (<a href="#FD6-mathematics-12-03946" class="html-disp-formula">6</a>) and (<a href="#FD5-mathematics-12-03946" class="html-disp-formula">5</a>) in <math display="inline"><semantics> <msup> <mi mathvariant="double-struck">R</mi> <mi>n</mi> </msup> </semantics></math>.</p> Full article ">
<p>Comparing methods to resolve (<a href="#FD4-mathematics-12-03946" class="html-disp-formula">4</a>) with the criterion space in the left part and decision space respectively in the right part.</p> Full article ">Figure 2
<p>A flow chart of searching a non-negative subset.</p> Full article ">Figure 3
<p>A convex set and efficient sets of (<a href="#FD3-mathematics-12-03946" class="html-disp-formula">3</a>), (<a href="#FD2-mathematics-12-03946" class="html-disp-formula">2</a>), (<a href="#FD6-mathematics-12-03946" class="html-disp-formula">6</a>) and (<a href="#FD5-mathematics-12-03946" class="html-disp-formula">5</a>) in <math display="inline"><semantics> <msup> <mi mathvariant="double-struck">R</mi> <mi>n</mi> </msup> </semantics></math>.</p> Full article ">
Open AccessFeature PaperArticle
The Assymptotic Invariants of a Fermat-Type Set of Points in
by
Mikołaj Le Van and Tomasz Szemberg
Mathematics 2024, 12(24), 3945; https://doi.org/10.3390/math12243945 - 15 Dec 2024
Abstract
In this paper, we compute asymptotic invariants—specifically, the Waldschmidt constants and the Seshadri constants—of a set of 31 points in , defined as the intersection points of a Fermat-type arrangement of planes.
Full article
(This article belongs to the Special Issue Advanced Algebraic Geometry and Applications)
Open AccessArticle
Data-Driven Voltage Control Method of Active Distribution Networks Based on Koopman Operator Theory
by
Zhaobin Du, Xiaoke Lin, Guoduan Zhong, Hao Liu and Wenxian Zhao
Mathematics 2024, 12(24), 3944; https://doi.org/10.3390/math12243944 - 15 Dec 2024
Abstract
The advent of large-scale distributed generation (DG) has introduced several challenges to the voltage control of active distribution networks (ADNs). These challenges include the heterogeneity of control devices, the complexity of models, and their inherent fluctuations. To maintain ADN voltage stability more economically
[...] Read more.
The advent of large-scale distributed generation (DG) has introduced several challenges to the voltage control of active distribution networks (ADNs). These challenges include the heterogeneity of control devices, the complexity of models, and their inherent fluctuations. To maintain ADN voltage stability more economically and quickly, a data-driven ADN voltage control scheme is proposed in this paper. Firstly, based on the multi-run state sensitivity matrix, buses with similar voltage responses are clustered, and critical buses are selected to downsize the scale of the model. Secondly, a linear voltage-to-power dynamics model in high-dimensional state space is trained based on the offline data of critical bus voltages, DGs, and energy storage system (ESS) outputs, utilizing the Koopman theory and the Extended Dynamic Mode Decomposition (EDMD) method. A linear model predictive voltage controller, which takes ADN stability and control cost into account, is also proposed. Finally, the effectiveness and applicability of the method are verified by applying it to an improved 33-bus ADN system. The proposed control method can respond more quickly and accurately to the voltage fluctuation problems caused by source-load disturbances and short-circuit faults.
Full article
(This article belongs to the Special Issue Intelligent Optimization and Control Modeling in Power and Energy System)
►▼
Show Figures
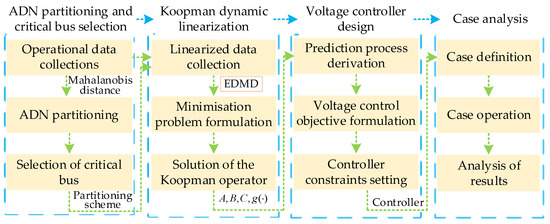
Figure 1
Figure 1
<p>Framework of the proposed voltage control strategy.</p> Full article ">Figure 2
<p>Schematic of linearization of voltage-power dynamics model.</p> Full article ">Figure 3
<p>Framework of voltage controller based on the high-dimensional model and MPC.</p> Full article ">Figure 4
<p>Multi-operation state sensitivity schematic. (<b>a</b>) Sensitivity of bus-2; (<b>b</b>) sensitivity of bus-5; (<b>c</b>) sensitivity of bus-15; (<b>d</b>) sensitivity of bus-20.</p> Full article ">Figure 5
<p>Schematic diagram of ADN partitioning and critical bus selection.</p> Full article ">Figure 6
<p>Prediction of RMSE in different lifting dimensions.</p> Full article ">Figure 7
<p>Variation of voltage magnitude at each critical bus under different control schemes. (<b>a</b>) Voltage at bus-15; (<b>b</b>) voltage at bus-21; (<b>c</b>) voltage at bus-26; (<b>d</b>) voltage at bus-32.</p> Full article ">Figure 8
<p>Variation of voltage magnitude at each critical bus under different control schemes. (<b>a</b>) Voltage at bus-15; (<b>b</b>) voltage at bus-21; (<b>c</b>) voltage at bus-26; (<b>d</b>) voltage at bus-32.</p> Full article ">Figure 9
<p>Variation of ADN voltage amplitude under different control schemes. (<b>a</b>) No-control; (<b>b</b>) K-VMPC-3; (<b>c</b>) K-VMPC-13; (<b>d</b>) S-MPC.</p> Full article ">
<p>Framework of the proposed voltage control strategy.</p> Full article ">Figure 2
<p>Schematic of linearization of voltage-power dynamics model.</p> Full article ">Figure 3
<p>Framework of voltage controller based on the high-dimensional model and MPC.</p> Full article ">Figure 4
<p>Multi-operation state sensitivity schematic. (<b>a</b>) Sensitivity of bus-2; (<b>b</b>) sensitivity of bus-5; (<b>c</b>) sensitivity of bus-15; (<b>d</b>) sensitivity of bus-20.</p> Full article ">Figure 5
<p>Schematic diagram of ADN partitioning and critical bus selection.</p> Full article ">Figure 6
<p>Prediction of RMSE in different lifting dimensions.</p> Full article ">Figure 7
<p>Variation of voltage magnitude at each critical bus under different control schemes. (<b>a</b>) Voltage at bus-15; (<b>b</b>) voltage at bus-21; (<b>c</b>) voltage at bus-26; (<b>d</b>) voltage at bus-32.</p> Full article ">Figure 8
<p>Variation of voltage magnitude at each critical bus under different control schemes. (<b>a</b>) Voltage at bus-15; (<b>b</b>) voltage at bus-21; (<b>c</b>) voltage at bus-26; (<b>d</b>) voltage at bus-32.</p> Full article ">Figure 9
<p>Variation of ADN voltage amplitude under different control schemes. (<b>a</b>) No-control; (<b>b</b>) K-VMPC-3; (<b>c</b>) K-VMPC-13; (<b>d</b>) S-MPC.</p> Full article ">
Open AccessArticle
On Symmetrical Sonin Kernels in Terms of Hypergeometric-Type Functions
by
Yuri Luchko
Mathematics 2024, 12(24), 3943; https://doi.org/10.3390/math12243943 - 15 Dec 2024
Abstract
In this paper, a new class of kernels of integral transforms of the Laplace convolution type that we named symmetrical Sonin kernels is introduced and investigated. For a symmetrical Sonin kernel given in terms of elementary or special functions, its associated kernel has
[...] Read more.
In this paper, a new class of kernels of integral transforms of the Laplace convolution type that we named symmetrical Sonin kernels is introduced and investigated. For a symmetrical Sonin kernel given in terms of elementary or special functions, its associated kernel has the same form with possibly different parameter values. In the paper, several new kernels of this type are derived by means of the Sonin method in the time domain and using the Laplace integral transform in the frequency domain. Moreover, for the first time in the literature, a class of Sonin kernels in terms of the convolution series, which are a far-reaching generalization of the power series, is constructed. The new symmetrical Sonin kernels derived in the paper are represented in terms of the Wright function and the new special functions of the hypergeometric type that are extensions of the corresponding Horn functions in two variables.
Full article
(This article belongs to the Special Issue Polynomials: Theory and Applications, 2nd Edition)
Open AccessArticle
Preview-Based Optimal Control for Trajectory Tracking of Fully-Actuated Marine Vessels
by
Xiaoling Liang, Jiang Wu, Hao Xie and Yanrong Lu
Mathematics 2024, 12(24), 3942; https://doi.org/10.3390/math12243942 - 14 Dec 2024
Abstract
In this paper, the problem of preview optimal control for second-order nonlinear systems for marine vessels is discussed on a fully actuated dynamic model. First, starting from a kinematic and dynamic model of a three-degrees-of-freedom (DOF) marine vessel, we derive a fully actuated
[...] Read more.
In this paper, the problem of preview optimal control for second-order nonlinear systems for marine vessels is discussed on a fully actuated dynamic model. First, starting from a kinematic and dynamic model of a three-degrees-of-freedom (DOF) marine vessel, we derive a fully actuated second-order dynamic model that involves only the ship’s position and yaw angle. Subsequently, through the higher-order systems methodology, the nonlinear terms in the system were eliminated, transforming the system into a one-order parameterized linear system. Next, we designed an internal model compensator for the reference signal and constructed a new augmented error system based on this compensator. Then, using optimal control theory, we designed the optimal preview controller for the parameterized linear system and the corresponding feedback parameter matrices, which led to the preview controller for the original second-order nonlinear system. Finally, a numerical simulation indicates that the controller designed in this paper is highly effective.
Full article
(This article belongs to the Special Issue Analysis and Applications of Control Systems Theory)
►▼
Show Figures
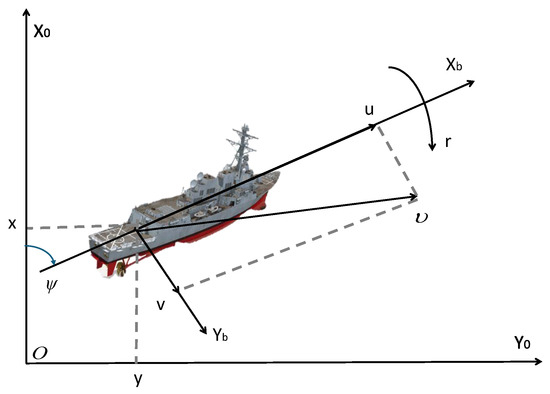
Figure 1
Figure 1
<p>The coordinate frames of the target model.</p> Full article ">Figure 2
<p>The block diagram for preview control design.</p> Full article ">Figure 3
<p>Surge Trajectory Tracking Response.</p> Full article ">Figure 4
<p>Sway trajectory tracking response.</p> Full article ">Figure 5
<p>Yaw trajectory tracking response.</p> Full article ">Figure 6
<p>Surge tracking error curves.</p> Full article ">Figure 7
<p>Sway tracking error curves.</p> Full article ">Figure 8
<p>Yaw tracking error curves.</p> Full article ">Figure 9
<p>Sum of surge tracking errors.</p> Full article ">Figure 10
<p>Sum of sway tracking errors.</p> Full article ">Figure 11
<p>Sum of yaw tracking errors.</p> Full article ">Figure 12
<p>Surge force control effort for trajectory tracking.</p> Full article ">Figure 13
<p>Sway force control effort for trajectory tracking.</p> Full article ">Figure 14
<p>Yaw moment control effort for trajectory tracking.</p> Full article ">Figure 15
<p>Sum of surge force.</p> Full article ">Figure 16
<p>Sum of sway force.</p> Full article ">Figure 17
<p>Sum of yaw moment.</p> Full article ">Figure 18
<p>Surge velocity tracking response.</p> Full article ">Figure 19
<p>Sway velocity tracking response.</p> Full article ">Figure 20
<p>Yaw angular velocity tracking response.</p> Full article ">Figure 21
<p>The entire physical processes.</p> Full article ">Figure 22
<p>Surge response under step input.</p> Full article ">Figure 23
<p>Sway response under step input.</p> Full article ">Figure 24
<p>Yaw response under step input.</p> Full article ">
<p>The coordinate frames of the target model.</p> Full article ">Figure 2
<p>The block diagram for preview control design.</p> Full article ">Figure 3
<p>Surge Trajectory Tracking Response.</p> Full article ">Figure 4
<p>Sway trajectory tracking response.</p> Full article ">Figure 5
<p>Yaw trajectory tracking response.</p> Full article ">Figure 6
<p>Surge tracking error curves.</p> Full article ">Figure 7
<p>Sway tracking error curves.</p> Full article ">Figure 8
<p>Yaw tracking error curves.</p> Full article ">Figure 9
<p>Sum of surge tracking errors.</p> Full article ">Figure 10
<p>Sum of sway tracking errors.</p> Full article ">Figure 11
<p>Sum of yaw tracking errors.</p> Full article ">Figure 12
<p>Surge force control effort for trajectory tracking.</p> Full article ">Figure 13
<p>Sway force control effort for trajectory tracking.</p> Full article ">Figure 14
<p>Yaw moment control effort for trajectory tracking.</p> Full article ">Figure 15
<p>Sum of surge force.</p> Full article ">Figure 16
<p>Sum of sway force.</p> Full article ">Figure 17
<p>Sum of yaw moment.</p> Full article ">Figure 18
<p>Surge velocity tracking response.</p> Full article ">Figure 19
<p>Sway velocity tracking response.</p> Full article ">Figure 20
<p>Yaw angular velocity tracking response.</p> Full article ">Figure 21
<p>The entire physical processes.</p> Full article ">Figure 22
<p>Surge response under step input.</p> Full article ">Figure 23
<p>Sway response under step input.</p> Full article ">Figure 24
<p>Yaw response under step input.</p> Full article ">
Open AccessArticle
Variational Information Principles to Unveil Physical Laws
by
D. Bernal-Casas and J. M. Oller
Mathematics 2024, 12(24), 3941; https://doi.org/10.3390/math12243941 - 14 Dec 2024
Abstract
This article demonstrates that the application of the variation method to purely information-theoretic models can lead to the discovery of fundamental equations in physics, such as Schrödinger’s equation. Our solution, expressed in terms of information parameters rather than physical quantities, suggests a profound
[...] Read more.
This article demonstrates that the application of the variation method to purely information-theoretic models can lead to the discovery of fundamental equations in physics, such as Schrödinger’s equation. Our solution, expressed in terms of information parameters rather than physical quantities, suggests a profound implication—Schrödinger’s equation can be viewed as a unique physical expression of a more profound informational formalism, inspiring new avenues of research.
Full article
Open AccessArticle
Spatial Position Reasoning of Image Entities Based on Location Words
by
Xingguo Qin, Ya Zhou and Jun Li
Mathematics 2024, 12(24), 3940; https://doi.org/10.3390/math12243940 - 14 Dec 2024
Abstract
The endeavor of spatial position reasoning effectively simulates the sensory and comprehension faculties of artificial intelligence, especially within the purview of multimodal modeling that fuses imagery with linguistic data. Recent progress in visual image–language models has marked significant advancements in multimodal reasoning tasks.
[...] Read more.
The endeavor of spatial position reasoning effectively simulates the sensory and comprehension faculties of artificial intelligence, especially within the purview of multimodal modeling that fuses imagery with linguistic data. Recent progress in visual image–language models has marked significant advancements in multimodal reasoning tasks. Notably, contrastive learning models based on the Contrastive Language-Image pre-training (CLIP) framework have attracted substantial interest. Predominantly, current contrastive learning models focus on nominal and verbal elements within image descriptions, while spatial locatives receive comparatively less attention. However, prepositional spatial indicators are pivotal for encapsulating the critical positional data between entities within images, which is essential for the reasoning capabilities of image–language models. This paper introduces a spatial location reasoning model that is founded on spatial locative terms. The model concentrates on spatial prepositions within image descriptions, models the locational interrelations between entities in images through these prepositions, evaluates and corroborates the spatial interconnections of entities within images, and harmonizes the precision with image–textual descriptions. This model represents an enhancement of the CLIP model, delving deeply into the semantic characteristics of spatial prepositions and highlighting their directive role in visual language models. Empirical evidence suggests that the proposed model adeptly captures the correlation of spatial indicators in both image and textual representations across open datasets. The incorporation of spatial position terms into the model was observed to elevate the average predictive accuracy by approximately three percentage points.
Full article
(This article belongs to the Section Mathematics and Computer Science)
►▼
Show Figures
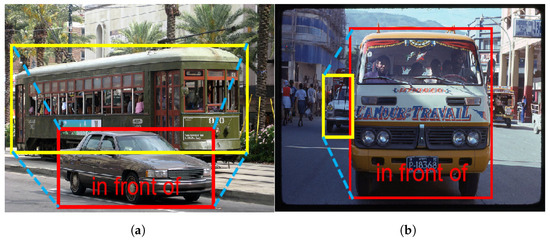
Figure 1
Figure 1
<p>The spatial position relationship between the prepositional phrase “in front of” and entities in an image. (<b>a</b>) The car is in front of the bus. (<b>b</b>) The bus is in front of the car.</p> Full article ">Figure 2
<p>Overall diagram of predictive and inference for locality noun. (<b>a</b>) describes contrastive pre-training learning, which constructs a contrastive learning matrix. (<b>b</b>) shows the processing of nouns for locality prediction.</p> Full article ">Figure 3
<p>Overall diagram of 7 spatial categories, including 71 types of spatial relations.</p> Full article ">Figure 4
<p>Comparison of location word prediction in different datasets: results on both random split (<b>a</b>) and zero-shot split (<b>b</b>)—model performance under different quantities of training data (10% 100% training set).</p> Full article ">Figure 5
<p>Thermodynamic diagram of the model’s attention to words. (<b>a</b>) Caption: the bus is <b>in front of</b> the car. (<b>b</b>) The phrase “in front of” is given more concentrated attention (the red area in the figure). (<b>c</b>) Caption: the person is <b>on</b> the airplane. (<b>d</b>) The word “on” is not the focus (the red area in the figure).</p> Full article ">Figure 6
<p>Prediction accuracy of single prepositions and prepositional phrases.</p> Full article ">
<p>The spatial position relationship between the prepositional phrase “in front of” and entities in an image. (<b>a</b>) The car is in front of the bus. (<b>b</b>) The bus is in front of the car.</p> Full article ">Figure 2
<p>Overall diagram of predictive and inference for locality noun. (<b>a</b>) describes contrastive pre-training learning, which constructs a contrastive learning matrix. (<b>b</b>) shows the processing of nouns for locality prediction.</p> Full article ">Figure 3
<p>Overall diagram of 7 spatial categories, including 71 types of spatial relations.</p> Full article ">Figure 4
<p>Comparison of location word prediction in different datasets: results on both random split (<b>a</b>) and zero-shot split (<b>b</b>)—model performance under different quantities of training data (10% 100% training set).</p> Full article ">Figure 5
<p>Thermodynamic diagram of the model’s attention to words. (<b>a</b>) Caption: the bus is <b>in front of</b> the car. (<b>b</b>) The phrase “in front of” is given more concentrated attention (the red area in the figure). (<b>c</b>) Caption: the person is <b>on</b> the airplane. (<b>d</b>) The word “on” is not the focus (the red area in the figure).</p> Full article ">Figure 6
<p>Prediction accuracy of single prepositions and prepositional phrases.</p> Full article ">
Open AccessArticle
Testing Spherical Symmetry Based on Statistical Representative Points
by
Jiajuan Liang, Ping He and Qiong Liu
Mathematics 2024, 12(24), 3939; https://doi.org/10.3390/math12243939 - 14 Dec 2024
Abstract
This paper introduces a novel chisquare test for spherical symmetry, utilizing statistical representative points. The proposed representative-point-based chisquare statistic is shown, through a Monte Carlo study, to considerably improve the power performance compared to the traditional equiprobable chisquare test in many high-dimensional cases.
[...] Read more.
This paper introduces a novel chisquare test for spherical symmetry, utilizing statistical representative points. The proposed representative-point-based chisquare statistic is shown, through a Monte Carlo study, to considerably improve the power performance compared to the traditional equiprobable chisquare test in many high-dimensional cases. While the test requires relatively large sample sizes to approximate the chisquare distribution, obtaining critical values from existing chisquare tables is simpler compared to many existing tests for spherical symmetry. A real-data application demonstrates the robustness of the proposed method against different choices of representative points. This paper argues that the use of representative points provides a new perspective in high-dimensional goodness-of-fit testing, offering an alternative approach to evaluating spherical symmetry in such contexts. By leveraging the flexibility of choosing the number of representative points, this method ensures more reliable detection of departures from spherical symmetry, especially in high-dimensional datasets. Overall, this research highlights the practical advantages of the proposed approach in statistical analysis, emphasizing its potential as a powerful tool in goodness-of-fit tests within the realm of high-dimensional data.
Full article
(This article belongs to the Special Issue Statistical Simulation and Computation: 3rd Edition)
Open AccessArticle
Enabling Sustainable Diffusion in Supply Chains Through Industry 5.0: An Impact Analysis of Key Enablers for SMEs in Emerging Economies
by
Chih-Hung Hsu, Jian-Cen Liu, Xue-Qing Cai, Ting-Yi Zhang and Wan-Ying Lv
Mathematics 2024, 12(24), 3938; https://doi.org/10.3390/math12243938 - 14 Dec 2024
Abstract
Industry 5.0 (I5.0) builds upon Industry 4.0 by emphasizing the role of workers in production processes and prioritizing socio-economic-environmental sustainability. It has been shown that I5.0 can enhance sustainability within supply chains (SCs). However, companies in emerging economies, especially small and medium-sized manufacturing
[...] Read more.
Industry 5.0 (I5.0) builds upon Industry 4.0 by emphasizing the role of workers in production processes and prioritizing socio-economic-environmental sustainability. It has been shown that I5.0 can enhance sustainability within supply chains (SCs). However, companies in emerging economies, especially small and medium-sized manufacturing enterprises (SMEs), which are crucial to developing economies, face challenges in implementing these concepts. These SMEs are in the early stages of adopting I5.0 to foster sustainability in their SCs and require urgent identification of key I5.0 enablers. Unfortunately, the current literature lacks research on this topic specifically within the context of SMEs in emerging economies. To bridge this gap, this study identifies the enablers of I5.0 that promote sustainability diffusion in SCs, using China’s SME manufacturing sector as a case study. The integrated framework for applying multiple criteria decision-making (MCDM) techniques in this study aims to assist decision-makers in evaluating different options and making optimal choices in a systematic and structured manner when faced with complex situations. The study employs the fuzzy Delphi method (FDM) to identify 15 key I5.0 enablers and categorize them into three clusters. Grey-DEMATEL is subsequently utilized to determine the causal relationships, rank the importance of the enablers, and construct an interrelationship diagram. This study found that ‘availability and functionality of resources’; ‘top management support, active participation, and effective governance’; ‘support from government, regulators, and financial resources’; and ‘introduction of safer and more efficient robotic systems for human–robot interaction and collaboration’ serve as the primary means of resolving issues. Overall, this study helps managers, practitioners, and policymakers interested in I5.0 applications to promote sustainability in the supply chain.
Full article
(This article belongs to the Special Issue Mathematics Applied to Manufacturing and Logistics Systems)
►▼
Show Figures
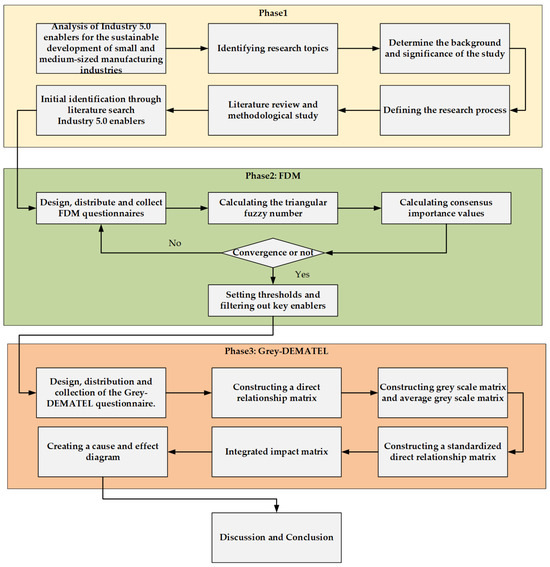
Figure 1
Open AccessArticle
Dynamics of Chronic Myeloid Leukemia Under Imatinib Treatment: A Study of Resistance Development
by
Irina Badralexi, Ana-Maria Bordei, Andrei Halanay and Ileana Rodica Rădulescu
Mathematics 2024, 12(24), 3937; https://doi.org/10.3390/math12243937 - 14 Dec 2024
Abstract
Chronic myeloid leukemia (CML) is a hematological disorder characterized by the abnormal proliferation of leukemic cells. This study aims to model the dynamics of leukemic and healthy cell populations in CML, considering the role of the immune system and the effects of treatment
[...] Read more.
Chronic myeloid leukemia (CML) is a hematological disorder characterized by the abnormal proliferation of leukemic cells. This study aims to model the dynamics of leukemic and healthy cell populations in CML, considering the role of the immune system and the effects of treatment with Imatinib. The model also addresses the development of treatment resistance in cells, following the Goldie–Coldman hypothesis. We employ a system of delay differential equations to simulate the interactions between leukemic cells, healthy cells, and the immune system under treatment. The results provide insights into the dynamic balance between leukemic cells, healthy cells, and immune responses, and the impact of developing resistance on treatment outcomes.
Full article
(This article belongs to the Section Mathematical Biology)
Journal Menu
► ▼ Journal Menu-
- Mathematics Home
- Aims & Scope
- Editorial Board
- Reviewer Board
- Topical Advisory Panel
- Instructions for Authors
- Special Issues
- Topics
- Sections & Collections
- Article Processing Charge
- Indexing & Archiving
- Editor’s Choice Articles
- Most Cited & Viewed
- Journal Statistics
- Journal History
- Journal Awards
- Society Collaborations
- Conferences
- Editorial Office
Journal Browser
► ▼ Journal BrowserHighly Accessed Articles
Latest Books
E-Mail Alert
News
Topics
Topic in
Algorithms, Axioms, Information, Mathematics, Symmetry
Fuzzy Number, Fuzzy Difference, Fuzzy Differential: Theory and Applications
Topic Editors: Changyou Wang, Dong Qiu, Yonghong ShenDeadline: 20 December 2024
Topic in
BDCC, Digital, Information, Mathematics, Systems
Data-Driven Group Decision-Making
Topic Editors: Shaojian Qu, Ying Ji, M. Faisal NadeemDeadline: 31 December 2024
Topic in
Future Internet, Information, J. Imaging, Mathematics, Symmetry
Research on Deep Neural Networks for Video Motion Recognition
Topic Editors: Hamad Naeem, Hong Su, Amjad Alsirhani, Muhammad Shoaib BhuttaDeadline: 31 January 2025
Topic in
Computers, Energies, Future Internet, Information, Mathematics
Research on Blockchain Technology for Peer-to-Peer (P2P) Energy Trading
Topic Editors: Pierre-Martin Tardif, Brahim El Bhiri, Bilal Abu-Salih, Kenji TanakaDeadline: 28 February 2025
Conferences
Special Issues
Special Issue in
Mathematics
Big Data Mining and Analytics with Applications
Guest Editors: Hongzhi Wang, Ye ChenDeadline: 20 December 2024
Special Issue in
Mathematics
Current Topics in Geometric Function Theory
Guest Editor: Nicoleta BreazDeadline: 20 December 2024
Special Issue in
Mathematics
Advanced Topics in Nonlinear Programming and Its Application in Robotic Control
Guest Editor: Ameer KhanDeadline: 20 December 2024
Special Issue in
Mathematics
Mathematics and Computer Science-Driven Innovations in Web3
Guest Editor: Sergey YablonskyDeadline: 20 December 2024
Topical Collections
Topical Collection in
Mathematics
Topology and Foundations
Collection Editors: Lorentz Jäntschi, Dušanka Janežič
Topical Collection in
Mathematics
Multiscale Computation and Machine Learning
Collection Editors: Yalchin Efendiev, Eric Chung
Topical Collection in
Mathematics
Theoretical and Mathematical Ecology
Collection Editor: Yuri V. Tyutyunov